Generative AI Security: Risks and Use Cases
Generative Artificial Intelligence (AI) is increasingly instrumental in helping organizations secure their systems, boosting the efficacy of cyber threat detection and response in ways that would have been considered science fiction a few years ago.
However, the progression of AI-based cybersecurity and data protection is essential for the effective defense of computer systems and networks from rapidly evolving cyber threats.
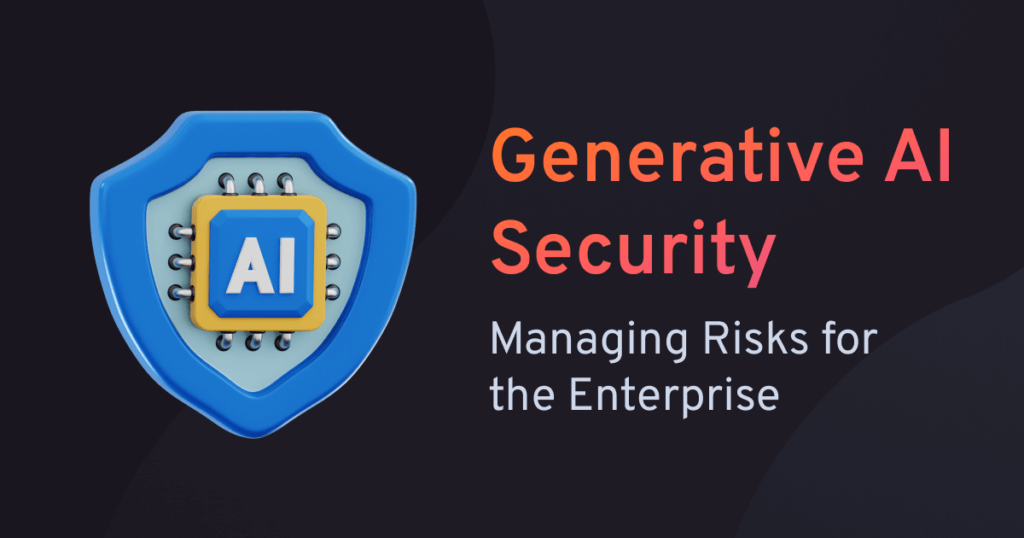
Table of Contents
- Introduction to Generative AI in Security
- Generative AI Integration Challenges
- Understanding the Generative AI Cybersecurity Market
- Top 5 Uses of Generative AI in Cyber Defense
- Addressing Security Risks and Threats in Generative AI Applications
- Global Insights: AI in the Security Sector
- The Future of Generative AI in Cybersecurity
- Conclusion
- Additional Resources
With a growing ecosystem of GPT-based tools, Generative AI makes it simpler for threat actors to conduct highly sophisticated and cost-effective attacks. Because threat actors themselves are now eagerly exploiting Generative AI to polish and sharpen their campaigns, it makes ultimate sense to use AI-based cybersecurity measures against them.
Enterprises must commit to proactively detecting and disabling those cyberattacks—and to optimize response and minimize damage when they do happen. For these purposes, Generative AI is invaluable, allowing enterprises to take an ideally preemptive approach to cybersecurity.
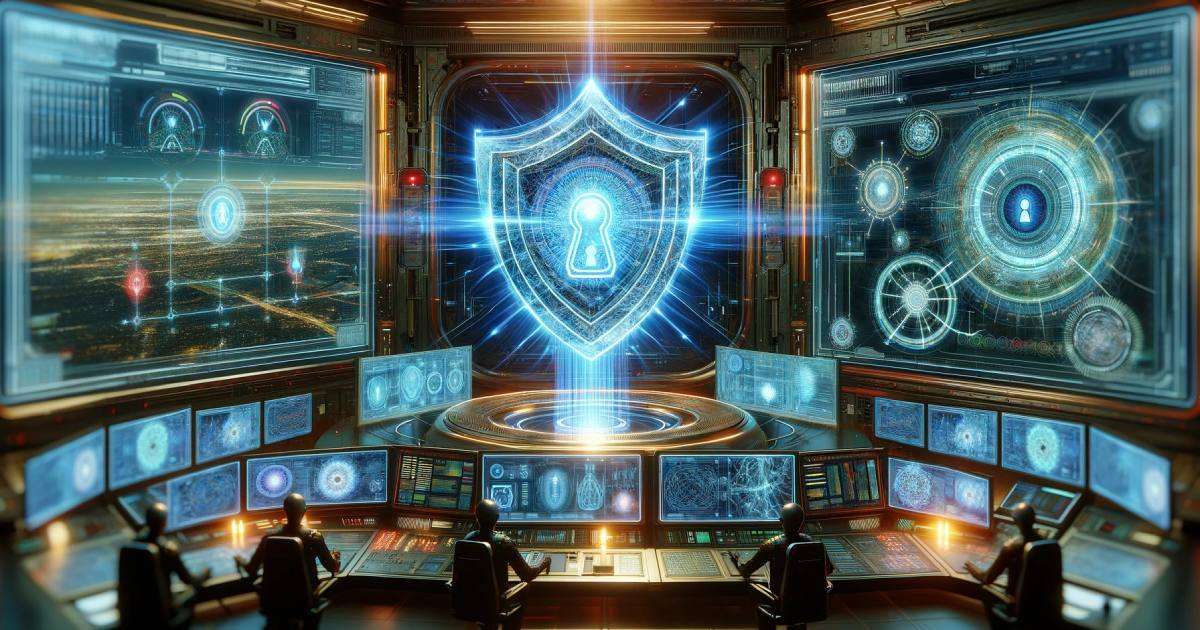
The value and risks of generative AI tools in pivoting from reactive to proactive threat detection can’t be overstated. By alerting teams to potential threats based on learned patterns, Generative AI empowers effective preemptive action, before a breach occurs. Generative AI’s ability to learn and replicate text patterns is a powerful resource that teams can now leverage to uncover cyber threats under construction, as well as unsuspected vulnerabilities among potential targets.
Enterprise Generative AI solutions like AiseraGPT can also gain a rapid understanding of security product documentation, for example, and help analysts activate tools quickly, with confidence in their efficacy.
To acquire knowledge of looming threats, large language models train on vast amounts of historical cybersecurity data. Today, rather than waiting to respond until threats actually launch—often so quickly that detection comes too late—security professionals can use Generative AI knowledge to anticipate threats before they materialize, including even while they are still under construction. This capability maximizes the functionality and investment value of enterprise security tools.
Moreover, the LLM security can serve as a pertinent case study in addressing and mitigating potential vulnerabilities inherent in AI-powered systems, providing crucial insights into the development of more secure AI applications.
One popular and relatively simple use case is to rely on AI to generate complex, unique passwords or encryption keys that are extremely difficult to guess or crack. Because weak or compromised credentials are convenient entry points for breaches, Generative AI offers a complementary layer of security for a cost-efficient investment.
By integrating these capabilities within an AI TRiSM framework, organizations can enhance transparency, accountability, and compliance, ensuring that the security measures enabled by Generative AI are not only effective but also align with best practices for responsible AI deployment.
Regarding cost-efficiency, as an AI-native system learns how to perform certain tasks, it helps security professionals to surface relevant information for making quick decisions. This accelerates analyst workflows by adding the ability to analyze data from different sources or modules.
Using Generative AI, teams can now shorten and condense time-intensive, tedious data analysis with confidence in its accuracy. Gaining the time and freedom to focus on new, challenging tasks also scales productivity and job satisfaction for the team. The ability to generate natural-language summaries of threat assessments and incidents further augments team output.
Although Generative AI is exciting and promising, it’s also important to be aware of the challenges that accompany it. Like any emerging technology, it must be approached and implemented cautiously and responsibly to mitigate risks or potential misuse.
Challenges to Integrating Generative AI Models into the Security Posture
AI as currently constituted is a resource-hungry technology. Generative AI training models consume high computational volumes, power, and storage. Smaller organizations may find this to be a significant consideration.
Additionally, there is the constant concern for attackers usurping or taking over AI resources for their nefarious purposes. Generative AI models and related tools are potentially vulnerable and accessible through open-source, inexpensive, cloud-based means.
Even as enterprises can apply Generative AI for cybersecurity purposes and mitigating risks, cybercriminals can appropriate Generative AI to create ingenious, dangerous attacks that are agile at evading even sophisticated cyber defenses.
Ethical considerations are another item of controversy. Issues of privacy, fairness, access management, and control over sensitive data have been the subject of serious public conversation as regards the types of data used by AI models in training datasets and the weight given to them in a diverse world.
Understanding the Generative AI Cybersecurity Market
Unquestionably, Generative AI is transforming cybersecurity, as data breaches and costs rise inexorably. Many security professionals are anxious that the Generative AI model will chronically risk giving cyber attackers the “upper hand” to sustain their offensives.
But enterprises are not passive about this danger They are aware of Generative AI’s potential in the right hands to improve and guide cyber defense. According to one report, 35% of CISOs are already employing AI for security applications; another 61% feel likely to use it during the next 12 months; 86% believe Generative AI will be useful in closing security skills gaps and dealing with talent shortages. Another 39% of CISOs plan to institute team training to acquaint employees with threats potentially posed by Generative Artificial Intelligence.
When it comes to geographies, North America unsurprisingly constitutes the leading market share for Generative AI cybersecurity resources. The soaring adoption of artificial intelligence technologies amid novel cyber threats explains the concern over gaps in security posture.
Europe is the second-largest market for artificial intelligence-based cybersecurity solutions, as cyber threats also multiply in that region. These spur increased government initiatives to promote the adoption of AI security technologies amid tight regulatory governance.
The Asia Pacific market is the fastest-growing region for AI-based cybersecurity solutions. Threats of cyberattacks in that region have been expanding as a consequence of the intensive adoption of AI technologies by businesses and governments.
The Middle East and Africa, although currently the smallest market for AI-based cybersecurity, is anticipated to grow at a rapid pace as businesses and governments accelerate their adoption of emerging technologies. If businesses are to thrive and flourish, it is vital that defense against cyberattacks expand concurrently to protect revenue and productivity for business growth in a sometimes politically and economically fragile region.
Top 5 Uses of Generative AI in Cyber Defense:
The integration of Generative AI in cybersecurity is revolutionizing how we protect sensitive information. Particularly Generative in banking, insurance, and healthcare, where confidentiality is paramount, Gen AI stands as a sentinel against data breaches and cyber threats. This technology not only fortifies defenses but also adapts and evolves, outsmarting even the most sophisticated cyber-attacks.
Dive into the use cases to explore the groundbreaking applications of Generative AI in securing our most vital industries and understand why it’s not just an innovation, but a necessity in the realm of cybersecurity.
1 - Creating Realistic Security Training Data
Generative AI in cybersecurity has two particularly relevant uses: to fashion sophisticated “white hat” attacks that are difficult to defend against and to inform the security training that familiarizes users with ways to spot and disable attacks. Generative AI in training applications empowers users to identify and report the following:
Believable phishing emails: Cybercriminals are skilled at using Generative AI to compose astoundingly realistic phishing emails of all types that fool users into clicking on malicious links or providing sensitive data, confidential data, or even funds.
Fake websites: Threat actors use Generative AI to fashion false websites that appear even under granular examination, to be legitimate. Users can be convinced to disclose personal information such as passwords and banking pins, or download harmful files that invade their systems.
Malicious code: Skilled hackers can employ Generative AI to produce code that targets vulnerabilities in computer systems.
AI can, however, implement new security solutions that are effective at detecting and neutralizing these attacks. Generative AI produces realistic training data for machine learning models which helps to create this data by testing new data and training users with the tactics of their adversaries, This exercise teaches them to spot realistic phishing emails, fake websites, and malicious code.
2 - Developing Advanced New Security Tools
Security experts are always at work developing the next generation of security tools that use Generative AI to detect and prevent attacks. Generative AI itself is a building block for creating tools that train machine learning models or assist security analysts in identifying and understanding potential attacks.
Machine learning is highly effective in using algorithms to identify patterns of malicious activity; deep learning-based anomaly detection systems use these algorithms to identify anomalies in network traffic that may indicate a cyberattack.
3 - Enhancing Existing System Security
There is no place for latency and legacy issues in today’s dynamic, unrelenting security environment. However, traditional cybersecurity resources can sometimes suffer from these latency issues, delaying identification and response to cyber threats. Generative AI’s real-time threat-hunting capabilities encourage rapid response times, reduce potential damage, and minimize the impact of cyber attacks.
4 - Simulating Sophisticated Cyber Attacks
Security analysts can use Generative AI to generate actual attack simulations to train themselves and test the effectiveness of security systems. Realistic scenarios sharpen our skills, and security experts can also use these tools to test security systems. Observing how security systems respond to distinct types of attacks educates users on their effectiveness and teaches them to spot weaknesses.
A strong security program must be heavily informed despite adversaries using cunning Generative AI tactics to fight back. Unleashing Generative AI as a defense resource is the smartest strategy an organization can take, and the sooner the better. Staging of safe simulations doesn’t need to wait for a real-world attack to demonstrate the threat modeling catastrophe. Rather, safe simulations show exactly how a threat actor could try to breach corporate vulnerabilities.
For example, an AI workflow platform can defend against spear phishing using Generative AI (targeted spear phishing cost companies an estimated $2.4 billion in 2021 alone). This workflow generated synthetic emails as examples of spear phishing messages. The AI model trained on that data learned to comprehend the intent of incoming emails by its natural language processing capabilities. Such NLP-based malware detection systems can use algorithms to identify malicious code in text files.
5 - Automating Essential Cybersecurity Operations
Too many cybersecurity professionals are still preoccupied with routine tasks, which distracts them from more critical, higher-value activities. Generative AI was created to automate repetitive, manual tasks like log analysis, threat hunting, and incident response.
Now, Generative AI frees up human experts to concentrate on more strategic and complex challenges. Machine learning-based intrusion detection systems also use ML algorithms to identify patterns of malicious activity. Here are seven security policy automation questions to focus on from Tim Woods, VP of Technology Alliances at Firemon:
- Does it improve people or process efficiency?
- How can it be measured?
- Does it result in greater consistency?
- Can it be quantified?
- How does it reduce risk?
- Are research skills aligned to achieve the defined objectives?
- Does it save the company money?
Addressing Security Risks and Threats in Generative AI Applications
The use of Generative AI in cybersecurity has the potential both to create sophisticated attacks that are difficult to defend against—and to develop new security strategies and tactics to defeat those.
It is sobering to realize that Generative AI is such a double-edged sword that it can arm both sides of the conflict! So as AI continues to develop, we can expect to see multiple innovative and effective ways to both attack and defend organizations. The ongoing development of Generative AI will hold the key to creating more workable, effective security solutions.
Ransomware exemplifies this endless attack versus defense model. One of the most feared and stubborn of cybercrimes, ransomware statistics reflect its frustrating power and persistence. Attacks increased 73% in Q2 2023 compared with the previous quarter. Fear that AI will give criminals the means to intensify their impact is ubiquitous.
The average ransomware payout in 2022 was $4.7M, and apprehension that threat actors will exploit new technology to reap even more lucrative outcomes is understandable. AI may enable threat actors to vastly speed up attack momentum and volume. Also, strategies are flexible and can respond quickly to opportunities.
The use of pure extortion and the threat of sensitive customer data or leaks rather than encrypting of data already represents such a tactical shift according to Infosecurity Magazine which also mentions a jaw-dropping 399% rise in cryptojacking in the first half of 2023 compared to 2022, reaching over 332 million hits. Researchers call this part of a trend to rely on lower-cost, less risky attack methods (e.g., stealing computing power to mine digital currency).
ChatGPT in the wrong hands can generate higher-quality impersonation capable of frustrating even the best hands-on training. It can mimic writing styles and corporate jargon convincingly enough to mislead even long-term trusted employees.
Generative AI can fortify those cybercriminals with a poor command of English by generating natural-sounding phraseology and wording for them to employ in their scams. It is important for organizations to be aware of these potential risks and to take steps to mitigate them. Denial only leads to disaster.
Global Insights: AI in the Security Sector and Use Cases
According to the Cost of a Data Breach Report 2023, published by IBM Security and Ponemon, data breach costs were dramatically lower (USD 1.76M versus USD 4.45M for the global average cost of a data breach) among companies using security AI and automation extensively to mitigate the financial impact of their breaches of business data.
The effect of extensive security AI and automation on the outcome of a breach is impressive and encouraging. Security AI and automation were shown to be important investments for an enterprise in reducing damage and minimizing time to identify and contain breaches.
Organizations that used these capabilities extensively in their approach experienced, on average, a 108-day shorter time to identify and contain the breach, along with those overall lower breach costs compared to organizations that didn’t use security AI and automation capabilities.
The Future of Generative AI in Cybersecurity
In McKinsey’s “The state of AI in 2023: Generative AI’s breakout year,” 79% of respondents admitted ‘some’ exposure to Generative AI, either for work or recreation. 22% said they regularly use it in their careers. In 2024, Generative AI is predicted to play an even more important critical role both in business and personal lives.
Adoption of Generative AI is certain to grow exponentially across various industries, as intelligent AI and machine learning-empowered systems fit themselves into our world. So the cybersecurity environment is set for challenges, opportunities, and innovations.
With Generative AI adoption and investment on the rise, McKinsey’s report revealed that many organizations are not addressing potential risks from secure use of Generative AI. Less than a third of respondents said they have measures in place to reduce the use of advanced AI technologies to mitigate cybersecurity risks. Establishing trust in AI through rigorous testing and transparency can help manage these risks effectively.
For the reasons mentioned above, Generative AI will probably make email less secure, a wake-up call to redraw lines of defense. Some believe that organizations will rely more on downstream security approaches such as Zero Trust, “a cybersecurity paradigm focused on data protection and the premise that trust in proprietary information is never granted implicitly but must be continually evaluated.”
Conclusion: The Evolving Role of Generative AI Security
Robust cybersecurity will always be the best defense. Even though some AI applications have fallen into the worrisome hands of malicious actors, we have the capabilities and technology to mount a nonstop defense against novel attacks and can rely on principled approaches to cybersecurity.
We can expect that Generative AI will transform the cybersecurity landscape in substantial ways. With the promise to advance the sophistication of security tools, boost threat intelligence, and pressure security operations centers toward excellence, we must focus on acquiring knowledge, supporting resilience, and committing to unite and stay one step ahead of our adversaries.
Defense in depth and layered security remain extremely important in defending against ransomware and whatever new attacks will appear on the horizon. Proactive strategies that can adapt to innovative attacks are particularly important.
AI-supercharged phishing emails, for example, are a signal for organizations to commit to advanced detection and response technologies. Manual human detection may be losing its reliability, but the human factor can still recognize an unauthorized user in your network or spot an active ransomware communication before it can infect.
Organizations can also rely on managed providers offering 24/7 detection and response as well as deploying proactive threat-hunting capabilities across your environments. By incorporating the TRAPS Framework, these providers can enhance their services with Transparency, Reliability, Accountability, Privacy, and Security, ensuring a defense-in-depth strategy that aligns with the highest standards of cybersecurity. This approach allows you to develop a comprehensive cybersecurity strategy at a fraction of the time and resources. Book a custom AI demo for your enterprise today!