Generative AI for Businesses and the Importance of Transparency in AI
Artificial Intelligence (AI) is about helping solve our problems—at least that’s how it has been conceptualized and presented by its creators. But humans, like all living things, have evolved to be suspicious of novelty, and for very good reasons which originated with survival itself.
It’s not surprising that many people view Generative AI in business as emulating human language, yet they do not consider it entirely trustworthy. Let’s get more details.
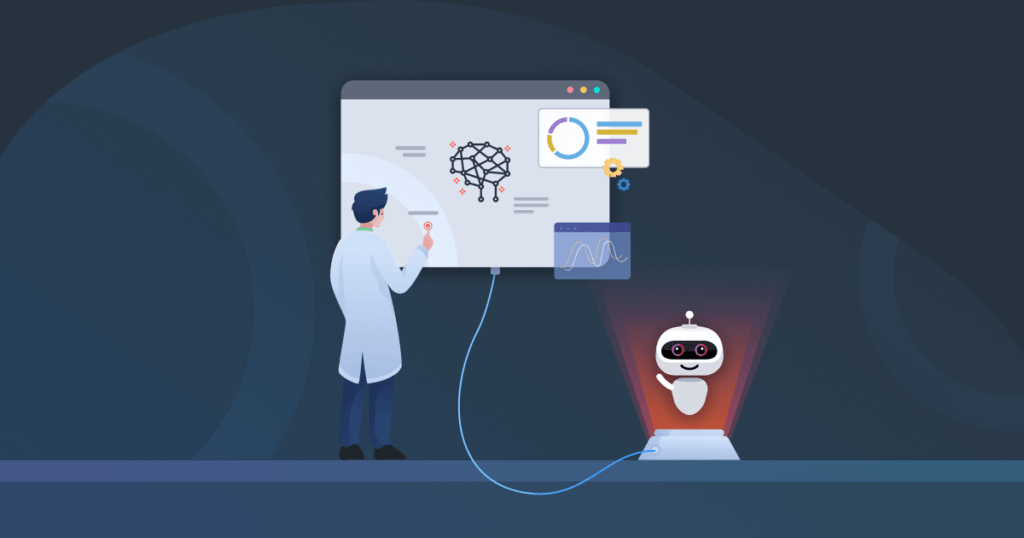
Generative AI seems to understand us, but at what level, and what does that mean? Will it someday redefine our idea of living versus non-living? How can we be sure that biases aren’t unintentionally engineered into AI systems? That ripple of mistrust we feel is inherent and inescapable.
According to Deloitte’s State of AI in the Enterprise report, 94% of business leaders surveyed agree that AI is critical to success over the next five years. As we invite this technology and its unique logic into our personal and professional lives, confiding our limitations and vulnerabilities; asking it to rescue us from failure or guide us toward success.
When we start using Generative AI in corporate environments, it’s crucial to recognize that these systems are trained with our data, reflecting our behaviors and biases. This process not only exposes our vulnerabilities but also amplifies the AI’s learning capabilities.
As we rely on AI to augment our analytical, reasoning, and decision-making skills, our approach must be tempered with a profound understanding of its implications. Our caution in deploying AI tools stems not from a mere technological concern but from a deeply rooted awareness of our human responsibility and the ethical considerations involved.
Generative AI for business: Building the bridge to trust through language
Language is the way our brains have evolved to communicate, and conversation is the form that language takes to connect us; to convey our thoughts and needs in an interaction. Language is the most intricate function of the human brain, and we are still at the beginning of exploring just how it happens.
Now we have innovated Generative AI and large language models (LLMs) which are machine learning models that use existing content like text, images, audio, video, and even code to generate content. The main purpose is to generate high-quality original content that seems real (that is, human-like). However, the use cases of Generative AI are not limited to content creation, even Generative AI is being used by Insurance companies for insurance case fraud detection. Also, Banks are using Generative AI for the same reason.
The AI system uses language and model training to learn and understand; to seek and absorb information, and to increase its insight and sophistication from each interaction and experience.
Because previous adventures into AI were not positive or deviated from accurate or expected answers based on training data, we now face the challenge of gaining their trust and assets in AI and in the organizations that employ it. Transparency is a step forward in the right direction to instilling that trust and has become indispensable to the growth and utility of Generative AI.
The Uses of Transparency in Generative AI
Overcoming distrust involves convincing people that an AI system, such as Generative AI for the IT department, embodies integrity, is incorruptible, and makes accurate, reliable decisions. People want to be assured that the system follows ethical principles defined during the design phase and aligns with the core principles of the organization. This requires AI transparency, observability, explainability, and responsibility.
Generative AI solutions in different industries including Generative AI in banking, insurance, and retail can produce an answer, but how did it arrive at that answer? Which criteria did it use? Does it embody the same values that we humans like to believe we operate by? The question of why machines think the way they do remains a challenge in systems that are not transparent.
Visibility and trust are major factors influencing the continued adoption and sustained usage of any AI platform in an enterprise. Analyzing historical data to achieve accuracy in prediction without ensuring the reliability of a recommendation is counter-productive.
So instilling transparency and responsibility will help organizations gain the benefits of Generative AI. The solution must convey confidence in the path it took to arrive at an answer and maintain a high level of accuracy. Transparent AI systems rely on numerous techniques to prove the validity of particular results. This helps address the “black box” syndrome, in which an AI system doesn’t provide sufficient information to make the output trustworthy. Lack of transparency is one of the key barriers to the adoption of autonomous AI today. It can lead to business and legal complexities that no enterprise wants to deal with.
A chatbot, for example, is based on inputs we build into it, and developers often pass along biases—consciously or unconsciously. AI may try to optimize the definition of fairness but there can be many interpretations of what “fair” is. For example, when determining creditworthiness: many complex factors can come into play.
These are debatable, complex, and controversial. Even when we believe we’ve programmed pristine, unbiased AI, how can we determine that we really have not built-in subtle beliefs? When AI systems have millions of parameters, it’s difficult to purge discriminating factors, and this creates distrust, often demonstrable under investigation and exposure in the media.
One reason why people might fear or distrust AI, is that AI technologies themselves can be hard to explain, says Evert Haasdijk, a senior manager Forensic at Deloitte and a renowned AI expert. Nevertheless, transparency is important to understand how significant the impact of a feature is and whether it is positive or negative. It’s vital to seek out insights that can continually improve the model and prevent biases from infecting systems.
The beginnings of AI regulatory responsibility
Generative AI models must provide evidence on how they arrive at a decision and can explain outcomes easily and comprehensively. Explaining the context and implications of an AI model and how it produces outcomes enables people to correct and retrain the models, which helps them trust the eventual results.
As the demand for transparency grows, so does the number of products aiming to help make models more transparent. Open source tools, such as Google’s What-If Tool and IBM’s AI Explainability 360 are addressing these doubts, and big tech companies are trying to embody them into their AI platforms. Microsoft has launched new interpretability tools in Azure Machine Learning; AWS released Amazon SageMaker Clarify, a tool for mitigating AI bias; and Google released Vertex Explainable AI for its Vertex AI MLOps platform. These efforts reflect the need for machine learning fairness metrics, helping data scientists understand and visualize the outcomes of their models.
“Glass Box” transparency is a healthy direction for generative AI and all users
Glass box models and visualizations are routes to making AI models reliably transparent to the user. In a glass box model, all features and model parameters are visible, and users also have access to the criteria used by a model to reach its prediction results and conclusion. Today, society is forcing AI in the direction of transparency as issues of fake news and other concerns have been exposed.
We can expect pressure for transparency from consumers to intensify; they will want to know why and how AI systems make decisions that influence their lives. Enterprise apps and services are expected to make decision-making transparent so the public can decide on whether generative AI-driven solutions are working with integrity.
This demands that AI/ML algorithms and solutions offer detailed reasoning and expose the root cause analysis they used in coming to conclusions. Think of transparency and observability as ongoing processes and goals rather than established and settled parameters. AI will always be a work in progress, just as the humans who design it will continue to be.