Introduction to the Role of AI in Knowledge Management
Knowledge management is a key part of modern enterprises. It’s the process of capturing, organizing, sharing, and using knowledge to get key insights, improve decision-making, and get ahead of the competition.
However, for most businesses, structured and unstructured data sits in many systems. This creates a messy environment that makes it hard to get to and manage. Traditional knowledge management systems can’t keep up with the variety of data, its availability, data quality, and most importantly the speed of knowledge creation.
What is AI Knowledge Management?
Artificial Intelligence is here to disrupt knowledge management. AI is not just a technology, it’s a force multiplier that automates the mundane, discovers insights, and makes knowledge available to everyone in the organization. With Enterprise AI Search and Neural Search, employees can find the correct information in seconds, increasing productivity and driving innovation.
As organizations adopt new technologies, the inclusion of AI in knowledge management will be critical to increasing operational efficiency, scalability, and user adoption. By automating routine tasks such as data collection, document indexing, and content creation, AI-driven knowledge management systems can significantly improve operational efficiency and allow employees to focus on more strategic roles.
From AI Annotation that improves knowledge categorization to Natural Language Processing (NLP) that organizes unstructured data, AI-driven knowledge management systems have the capabilities to address the existing challenges.
Here, we’ll look at AI in knowledge management, its features, benefits, challenges, and future. For IT leaders, knowledge managers, and business strategists, it’s essential to get ahead of the curve.
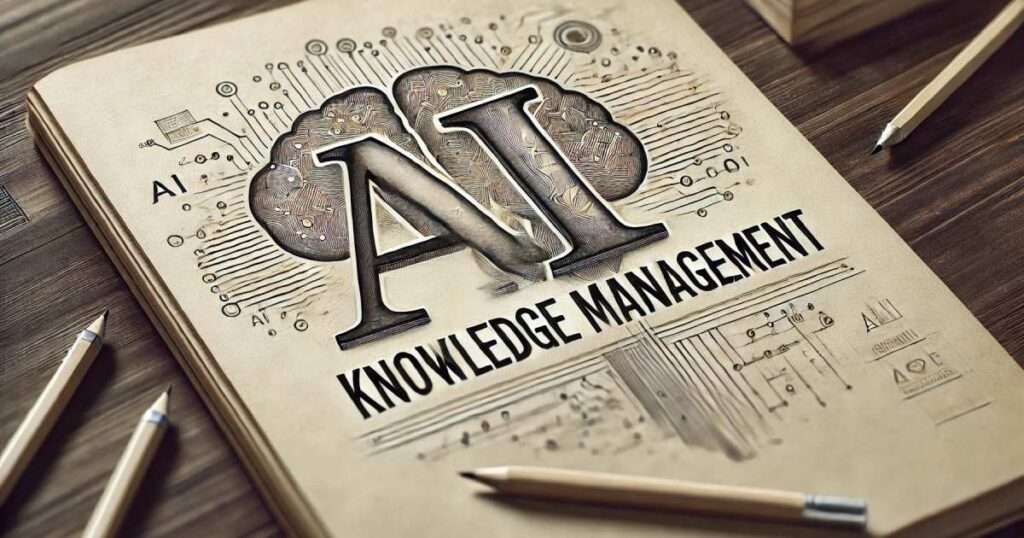
Understanding Advanced Knowledge Management Systems
AI in Knowledge Management (KM) refers to the application of Artificial Intelligence to enhance the way organizations capture, organize, and utilize knowledge. A well-implemented knowledge management strategy leverages AI to streamline knowledge capture and organization, improving business outcomes through better decision-making.
By leveraging technologies such as Enterprise AI Search, Neural Search, and Natural Language Processing (NLP), AI interprets unstructured and fragmented data into actionable knowledge. This further helps in driving innovation and better decision-making in organizations.
Core Components of AI Knowledge Management Systems
- Enterprise AI Search: AI elevates search as a service capability by understanding the context of queries and providing relevant results across vast, heterogeneous datasets. This ensures employees can retrieve precise information quickly, even from unstructured sources like emails or ticket comments.
- Contextual Knowledge Recommendations: AI systems can analyze ongoing workflows, such as resolving customer tickets, and provide contextual recommendations for the next best actions. This reduces labor-intensive processes, as agents can directly use curated solutions without manual copying or editing.
- Knowledge Creation from Ticket Comments: AI tools extract valuable insights from ticket comments, which are often overlooked in traditional systems. These tools can identify resolution notes and generate comprehensive knowledge articles, enriching the knowledge repository and ensuring that the collective experiences of agents are utilized effectively.
- AI Annotation and Automated Tagging: By categorizing and tagging content automatically, AI ensures that knowledge is organized and easily discoverable. This eliminates the need for manual sorting, saves time, and improves consistency.
- Similarity Checks for Quality Control: AI-powered similarity checks validate newly created knowledge against existing repositories to avoid redundancy. Non-duplicate documents are committed automatically, while duplicates are flagged for manual review, ensuring a high-quality knowledge base.
How AI Transforms Knowledge Management
AI-driven KM systems are more than just repositories; they transform knowledge management into dynamic ecosystems that learn, adapt, and grow with organizational needs. They:
- Identify patterns and generate new insights by clustering related tickets and cases.
- Reduce operational bottlenecks by providing instant, contextual solutions to users.
- Enable continuous improvement of knowledge repositories through automated ingestion and refinement processes.
By integrating these capabilities, AI enables organizations to transition from static knowledge management to intelligent, responsive systems that drive efficiency, collaboration, and growth.
Key Features of AI Knowledge Management Systems
AI-powered Knowledge Management (KM) systems come equipped with advanced features that enable organizations to optimize their information workflow systems and enhance decision-making. These systems suggest relevant knowledge resources based on user preferences, providing personalized insights and automating tasks. These features go beyond traditional KM systems, offering automation, contextual intelligence, and scalability. Below are the key capabilities:
1. Intelligent Search and Retrieval
AI search engines, like Enterprise AI Search, use Neural Search and NLP to deliver precise, context-aware results. Employees no longer need to sift through endless documents; instead, they can retrieve relevant information instantly, saving time and reducing frustration.
2. Automated Content Tagging and Categorization
Manual tagging can be inconsistent and time-consuming. AI automates this process by analyzing content and assigning appropriate tags, ensuring a consistent and structured knowledge repository.
3. Natural Language Processing for Unstructured Data
Unstructured data—like ticket comments, emails, and meeting notes—comprises the majority of enterprise information. AI systems equipped with NLP can interpret, categorize, and extract insights from this data, converting it into actionable knowledge.
4. Personalized Content Recommendations
AI provides tailored recommendations based on user behavior, roles, and past interactions. For example, in an IT service environment, AI might recommend troubleshooting guides or similar resolved cases, enabling agents to resolve issues faster.
5. Knowledge Discovery and Insight Generation
AI identifies patterns and relationships within data that may not be immediately apparent. By clustering similar tickets or cases, it facilitates the discovery of new insights and the creation of comprehensive knowledge articles.
6. Predictive Analytics for Knowledge Usage Trends
AI-powered KM systems can analyze usage trends and predict knowledge needs. For instance, they might highlight gaps in the repository or anticipate seasonal spikes in certain types of queries, enabling proactive content creation.
7. Contextual Assistance and Actionable Suggestions
Advanced AI platforms offer contextual assistance by analyzing ongoing workflows. For example: If an agent is working on a case, the system might suggest pre-curated responses or recommend creating a knowledge article if no existing solution is found. This reduces manual effort, ensures relevance, and empowers agents to deliver faster resolutions.
8. Duplicate Detection and Knowledge Validation
Duplicate knowledge can clutter repositories and create confusion. AI systems use similarity-check algorithms to compare newly created content with existing documents, flagging duplicates for review and maintaining a high-quality repository.
Benefits of AI-Driven Knowledge Management
AI-powered Knowledge Management (KM) systems excel in managing organizational knowledge, and addressing traditional challenges while delivering significant operational advantages. Here are the five key benefits:
1. Improved Knowledge Accessibility and Retrieval
AI-driven KM systems use advanced tools like Enterprise Search to ensure employees can quickly access the right information. By leveraging these technologies AI enables precise and context-aware retrieval of data, reducing the time spent searching through large repositories.
2. Enhanced Employee Productivity and Decision-Making
With AI automating repetitive tasks and providing actionable recommendations, employees can focus on higher-value activities. For example:
- Customer support agents can resolve tickets faster with AI-suggested solutions tailored to the case’s context.
- Decision-makers gain insights through predictive analytics, enabling faster and more informed business strategies.
3. Knowledge Retention and Loss Prevention
AI ensures that valuable institutional knowledge is captured and preserved. By extracting insights from ticket comments, historical data, and existing knowledge repositories, AI prevents the loss of critical information due to employee turnover or incomplete documentation.
4. Scalability for Growing Organizational Needs
As organizations grow, so does the complexity of their knowledge management requirements. AI-powered systems are designed to scale effortlessly, handling larger datasets, diverse information sources, and increasing user demands while maintaining performance and reliability.
5. Reduction in Operational Inefficiencies
By automating manual processes like content tagging, duplication checks, and clustering, AI eliminates inefficiencies that slow down workflows. This leads to faster processes, reduced errors, and optimized resource utilization.
By addressing accessibility, productivity, retention, scalability, and inefficiency, AI-driven KM systems empower organizations to operate more effectively and adapt to future challenges. These systems not only improve day-to-day operations but also ensure long-term organizational success.
Challenges and Ethical Considerations
While AI-powered Knowledge Management (KM) systems offer immense potential for managing organizational knowledge, organizations face certain challenges that can impede their effectiveness. These challenges, however, can be addressed with the right AI solutions. Below are some key hurdles:
Handling Unstructured and Incomplete Data
Unstructured data, such as ticket comments, emails, and meeting notes, often contains critical insights but is difficult to process using traditional KM systems. Additionally, incomplete or inconsistent data can result in low-quality knowledge generation.
Data Privacy and Compliance
AI-powered KM systems often handle sensitive data, raising concerns about privacy and regulatory compliance. Failure to address these concerns can lead to trust issues and potential legal risks.
Integration with Legacy Systems
Many organizations struggle to integrate AI-powered KM solutions with their existing platforms, such as CRMs or service desks. Legacy systems often lack the flexibility to support AI tools, creating silos of information.
Cost and Resource Investments
Implementing AI-driven KM systems often requires significant upfront investments in infrastructure, tools, and training. Organizations may also need specialized talent to manage AI models, which can strain budgets and resources, particularly for small and medium-sized businesses.
Ethical Implications of AI
As AI becomes integral to Knowledge Management, concerns about fairness, transparency, and accountability arise. Issues such as bias in AI recommendations, lack of human oversight, and potential misuse of sensitive data can hinder trust in AI systems.
How to Implement AI-Driven Knowledge Management Systems
Implementing AI in Knowledge Management (KM) requires a strategic approach to ensure successful adoption and measurable outcomes. Integrating AI technologies can significantly enhance knowledge management practices, making them more efficient, adaptable, and compliant. Below is a step-by-step guide
Step 1: Assess Current KM Capabilities
Begin by identifying gaps in your current knowledge management processes. Evaluate your repository’s completeness and your team’s ability to retrieve information efficiently. This diagnostic step ensures you have a clear roadmap for improvement.
Step 2: Organize and Clean Knowledge Repositories
Before introducing AI, ensure your existing knowledge base is well-organized. Eliminate redundant or outdated information and structure your data for optimal AI processing.
Step 3: Choose the Right AI Tools
Select AI platforms that align with your organization’s needs. For example:
- Tools integrate seamlessly with CRMs and service desks.
- Ensure scalability and flexibility to adapt to future needs.
Step 4: Train AI Models for Specific Use Cases
AI is most effective when tailored to your organization. Use historical data like ticket comments or knowledge articles to train the system to generate actionable insights.
Step 5: Monitor and Optimize Performance
AI implementation isn’t a “set-it-and-forget-it” process. Regularly review AI-generated knowledge, track performance metrics, and refine models to improve relevance and accuracy over time.
Step 6: Prioritize Ethical Use and Compliance
Adopt Responsible AI practices to ensure transparency, fairness, and compliance with regulations like GDPR. This builds trust and mitigates risks.
Real-World Applications of AI Knowledge Management
AI-powered Knowledge Management (KM) systems are revolutionizing how organizations manage knowledge and handle information across industries. Here are some real-world applications and success stories showcasing their impact:
1. IT and Customer Support
AI streamlines ticket resolution and enhances support workflows by:
- Generating actionable knowledge from historical ticket comments.
- Suggesting pre-curated responses for active cases, reducing resolution times.
Example: AI-powered platforms empower IT teams to handle high ticket volumes with contextual recommendations, ensuring faster resolutions and improved user satisfaction.
2. Healthcare
AI in KM helps healthcare organizations manage vast amounts of medical data, including patient records and research findings:
- Enables secure access to medical knowledge while maintaining compliance with regulations like HIPAA.
Example: Generating structured insights from unstructured clinical notes improves diagnostics and treatment planning.
3. Financial Services
AI-powered KM supports compliance and enhances decision-making in heavily regulated environments:
- Identifies knowledge gaps and generates new content for evolving regulations.
Example: AI generates knowledge articles on fraud detection, ensuring financial institutions stay ahead of threats.
4. Retail and E-Commerce
Retailers use AI to manage product knowledge, enhance customer interactions, and streamline inventory management:
- Provides instant, accurate answers to customer queries through chatbots and AI-driven search.
Example: AI helps e-commerce platforms recommend solutions for common customer issues, reducing cart abandonment rates.
5. Education and Training
AI-powered KM simplifies knowledge-sharing in academic and corporate settings:
- Generates training materials from existing documents, ensuring employees or students have access to updated information.
Example: Automating knowledge creation for onboarding new employees saves time and ensures consistency.
The Future of Knowledge Management with AI
As technology continues to evolve, the future of AI in Knowledge Management (KM) promises even greater advancements. Here are some emerging trends and predictions shaping the next decade of KM:
1. Generative AI for Knowledge Creation
AI systems are becoming increasingly capable of creating knowledge articles, summaries, and solutions autonomously. Future systems will:
- Use Generative AI to fill gaps in knowledge repositories proactively.
- Enhance collaboration by generating dynamic content tailored to team needs.
2. AI-Powered Collaboration Ecosystems
AI will foster real-time knowledge sharing by:
- Integrating with team collaboration tools like Slack, Microsoft Teams, and Google Workspace.
- Providing instant access to relevant knowledge during discussions or problem-solving sessions.
3. Personalized Knowledge Delivery
AI will move beyond role-based recommendations to deliver hyper-personalized knowledge experiences:
- Predicting user needs based on behavior, preferences, and historical queries.
- Delivering contextual insights in real-time, reducing information overload.
4. Enhanced Trust and Ethical AI Practices
As ethical concerns grow, organizations will prioritize building Responsible AI frameworks. This includes:
- Transparent algorithms for decision-making.
- Bias mitigation to ensure fairness in AI-driven recommendations.
5. Autonomous Knowledge Evolution
AI systems will become self-improving, learning from interactions and continuously optimizing:
- Identifying and resolving gaps in knowledge repositories without manual intervention.
- Enhancing predictive capabilities for emerging knowledge needs.
6. Domain-Specific AI Solutions
The future will see more tailored AI KM solutions catering to niche industries:
- AI systems designed for healthcare will prioritize compliance and patient privacy.
- Retail-focused systems will emphasize customer personalization and inventory insights.
The Road Ahead
The future of Knowledge Management (KM) lies in AI systems that are not only intelligent but also adaptive, ethical, and scalable. Central to this evolution is agentic AI and AI agents, which redefine how organizations approach knowledge—making it a core driver of efficiency and innovation. Here’s a glimpse into what lies ahead:
Adaptive and Contextual Knowledge Systems: AI-driven KM platforms will become more adaptive, understanding the context of user queries and delivering highly relevant information instantly. Enterprise AI agents, with their advanced reasoning capabilities, will:
- Anticipate the next steps in resolving a ticket or completing a task, enhancing user interactions by providing proactive support.
- Generate actionable knowledge articles from ticket clusters or historical interactions, autonomously creating and refining content based on user feedback and needs.
Unified Knowledge Ecosystems: The KM systems of tomorrow, powered by AI agents, will unify data from multiple platforms, eliminating silos. These agents will seamlessly integrate with CRMs, service desks, or team collaboration tools to:
- Provide a single source of truth across departments, ensuring consistent and accurate information is accessible.
- Enable seamless access to knowledge, empowering employees to make better decisions with integrated, real-time data.
Proactive Knowledge Creation and Maintenance: Shifting from a reactive to a proactive paradigm, agentic AI will:
- Continuously analyze new data to identify knowledge gaps, using AI agents to auto-generate and update content, ensuring repositories stay relevant and up-to-date.
- Employ advanced algorithms to monitor and adapt knowledge bases in real-time, maintaining the accuracy and usefulness of the information provided.
Responsible AI Practices at the Core: Ethics and transparency will be central to future KM systems, with a focus on deploying agentic AI solutions that:
- Build trust by ensuring fair and unbiased recommendations designed with a commitment to ethical AI practices.
- Adhere to strict data privacy regulations, fostering confidence among users and customers, with transparency in how data is used and protected.
Scalable Solutions for Growing Needs: As enterprises grow, their KM requirements expand exponentially. Agentic AI and AI agents are designed to:
- Handle vast datasets without compromising performance, adapting to increased demands with scalable solutions.
- Scale effortlessly to meet the needs of global, hybrid, and remote teams, ensuring efficient knowledge management across diverse organizational structures.
Organizations looking to embrace these advancements need a trusted partner to guide them through the evolving landscape of AI-driven Knowledge Management. This is where Aisera excels. With a proven track record of innovation, Aisera’s platform combines adaptive AI, seamless integrations, and advanced automation to help businesses transform their knowledge repositories into dynamic, actionable resources.
By leveraging features such as intelligent contextual assistance, automated knowledge creation, and proactive content updates, Aisera ensures that companies stay ahead of the curve. Its solutions are designed not only to enhance operational efficiency but also to foster innovation and long-term growth, making it an indispensable ally for enterprises navigating the future of Knowledge Management.
Conclusions
AI-powered Knowledge Management has transformed from a futuristic concept to a present-day necessity for organizations seeking to scale, innovate, and maintain competitiveness. Today’s advanced KM solutions enable enterprises to evolve from static information repositories into dynamic, actionable resources that drive operational efficiency and foster growth. These technologies are essential for enhancing customer support, streamlining internal processes, and future-proofing business strategies.
Embracing intelligent KM platforms allows organizations to harness the full potential of their collective knowledge, making it easier to meet current challenges and anticipate future needs. As businesses continue to navigate a rapidly changing landscape, adopting AI-driven knowledge management is not just an option; it’s a strategic imperative for sustained success and resilience.
Ready to explore the transformative impact of AI on your knowledge management strategies? Take the first step towards a smarter, more efficient future by considering the integration of advanced AI solutions in your operations. Schedule an AI demo with Aisera today to see how our AI-driven solutions can revolutionize your Knowledge Management strategy and drive your organization toward success.