Top of the CLASS: Benchmarking LLM Agents on Real-World Enterprise Tasks
Aisera AI Agents
GPT-4o
Claude 3.5 Sonnet
Gemini 1.5 Pro
Learn why domain-specific AI agents deliver business value better than general-purpose AI agents built on foundational models.
Accepted at the ICLR 2025 Workshop on Building Trust in LLMs and LLM Applications
Download Report
Introducing the CLASSic Framework
Aisera, the leading Agentic Al platform provider to Fortune 500 enterprises led a study to introduce the CLASSic framework – a holistic approach to evaluating enterprise Al agents across five key dimensions:
Results and Analysis
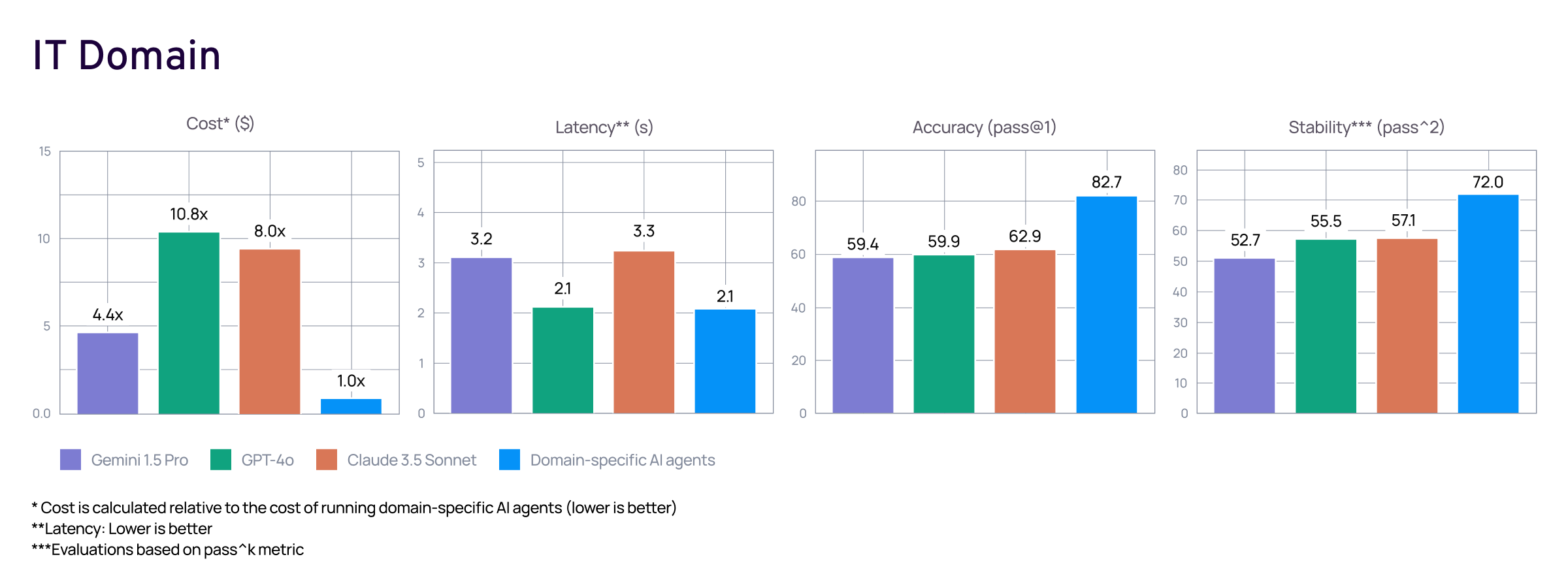
Based on the results for the IT domain, specialized domain-specific AI agents emerges as the performance leader in enterprise IT operations, dramatically outperforming AI agents built directly on foundation models. With an industry-leading accuracy of 82.7% and the highest stability score of 72%, domain-specific AI agents delivers significantly more reliable results while operating at just a fraction of the cost. This superior performance, combined with the fastest response time of 2.1 seconds, demonstrates the unique optimization that domain-specific AI agents bring to enterprise IT environments, where speed, reliability, and cost-efficiency are mission-critical.
Overall key findings include:
- Domain-Specific Advantage: Domain-specific AI agents excel in accuracy and stability, outperforming agents built directly on top of general-purpose models by leveraging domain specialization. AI agents built over general-purpose LLMs often lack enterprise-specific knowledge (e.g., understanding “What does Error 5 mean?” requires product-specific insights), requiring additional fine-tuning for comparable results.
- Cost-Performance Trade-offs: While Agents built on Claude achieves second-best accuracy, it does so at higher operational costs, highlighting the need for cost-efficient benchmarks and balanced evaluations beyond just accuracy.
- Latency and Usability: Agents built on GPT-4o on a dedicated Azure endpoint provided the fastest responses, closely followed by domain-specific AI Agents. Further improvements can be achieved with caching and parallelization.
- Security: Agents built on frontier models like Gemini and GPT-4o were more vulnerable to prompt manipulation attacks than specialized systems such as domain-specific AI agents. Adversarial testing and standardized protocols are critical for building resilient AI systems.
For each of the general-purpose frontier models, we adapt them to the task of workflow selection using the popular ReAct framework for agentic AI. By comparing domain-tuned agents to off-the-shelf models, we illustrate the trade-offs between specialized models and generic systems1.
1This study compares AI agents built on domain specific application architectures with AI agents built on frontier large language models and does not offer direct comparison between LLMs.
AI Agents That Deliver Business Value: Evaluating What Truly Matters
As enterprises embrace AI agents to boost efficiency and tackle complex tasks, traditional accuracy-based benchmarks fall short.
The CLASSic framework, a first-of-its-kind developed by Aisera, is a holistic evaluation methodology that assesses Cost, Latency, Accuracy, Stability, and Security for enterprise AI Agents.
Applying the CLASSic framework to real-world data from five industries, shows that purpose-built, domain-specific AI agents outperform foundational models across multiple evaluation dimensions.
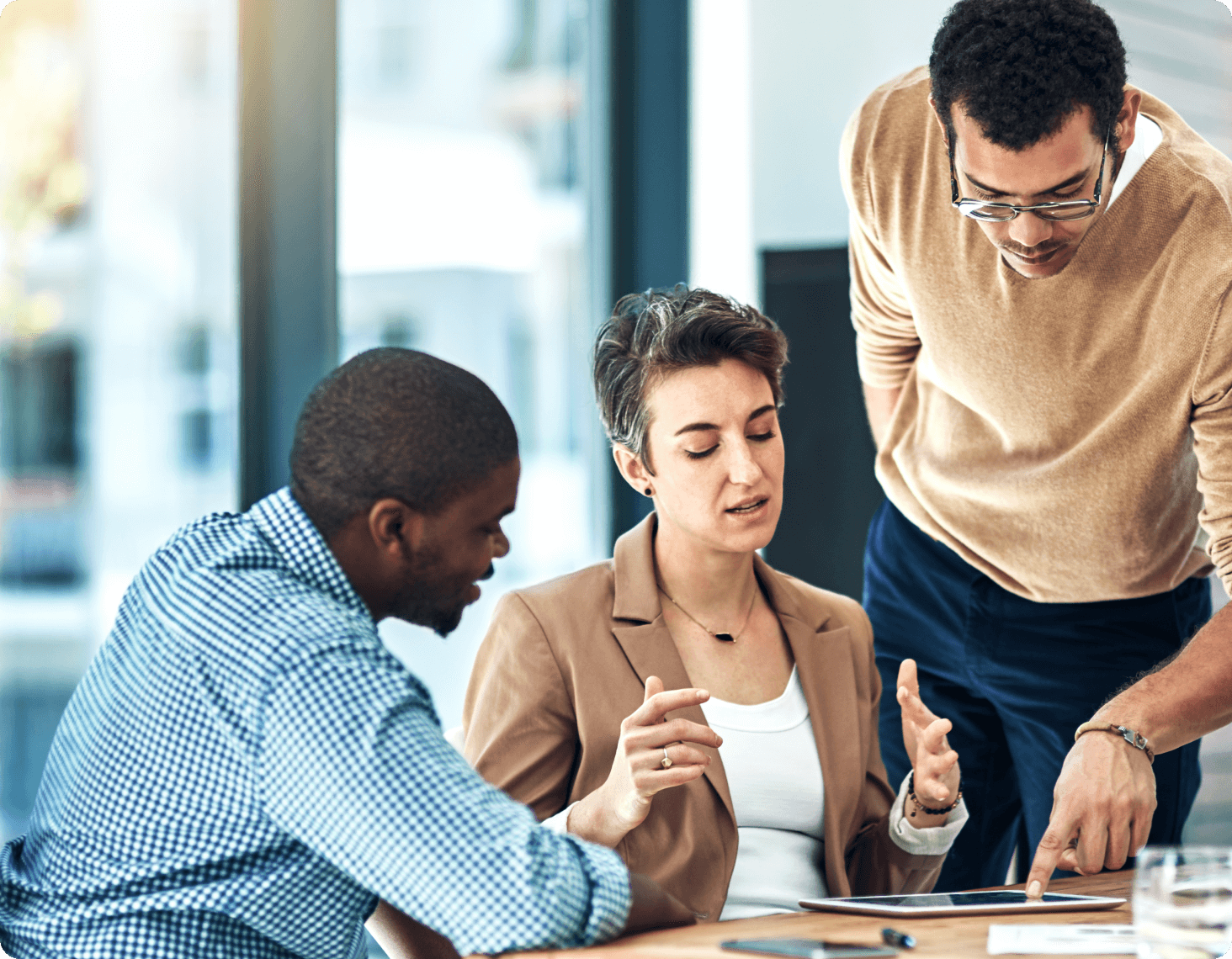
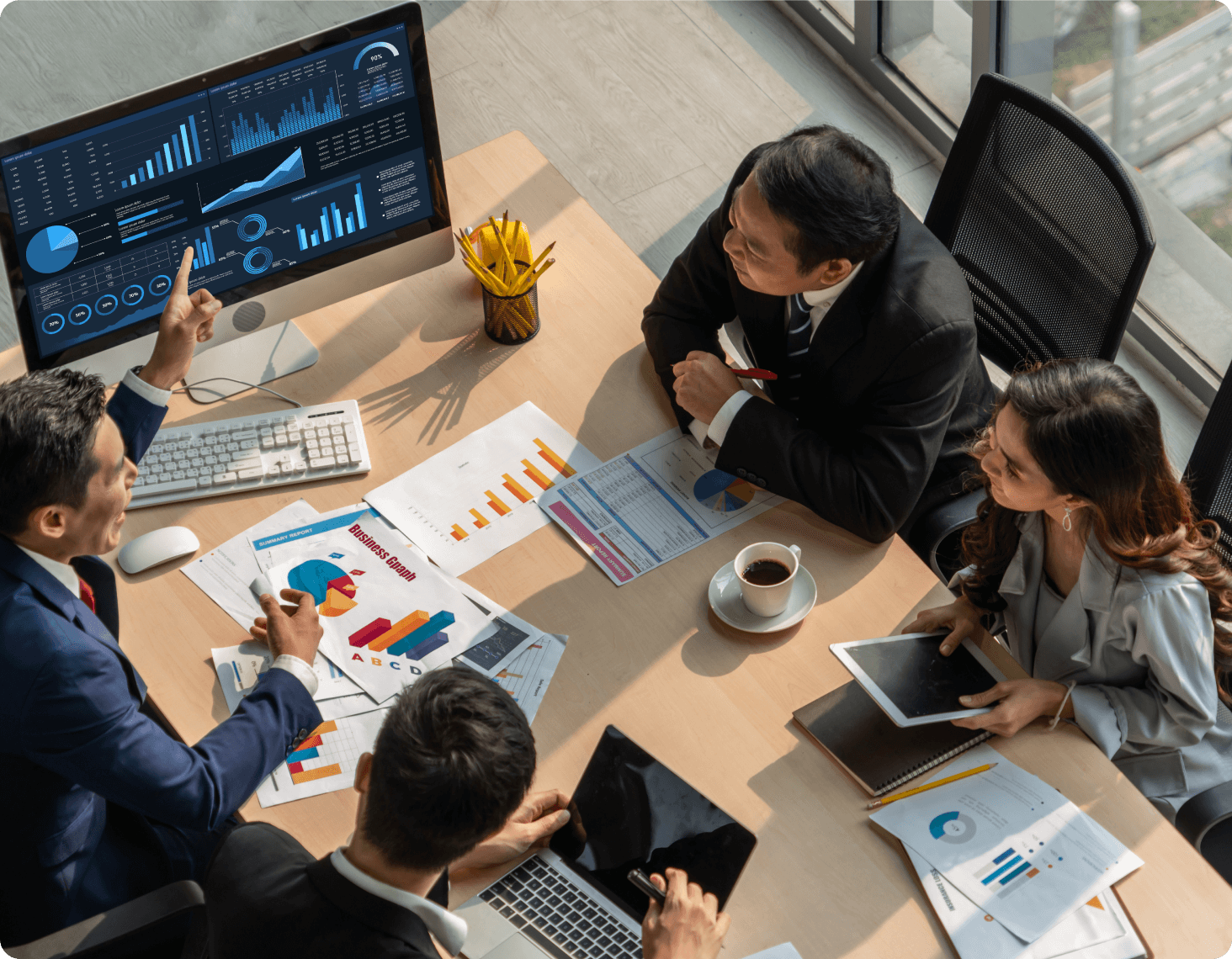
Datasets
Thousands of real-world user-chatbot interactions were selected across seven industries, capturing domain-specific jargon, workflows, and multi-turn dialogues. Each domain presents unique challenges—such as handling sensitive patient data (Healthcare IT) or navigating complex financial compliance (Financial HR)—ensuring agents face real-world variability and avoid overfitting to narrow test sets.
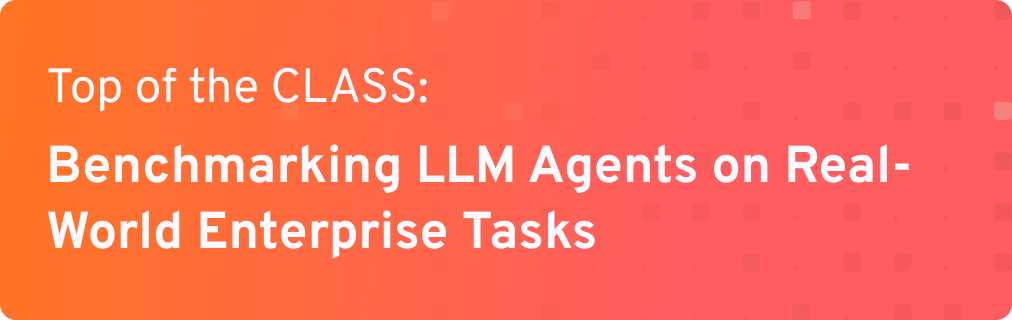
Get your copy of the benchmarking report
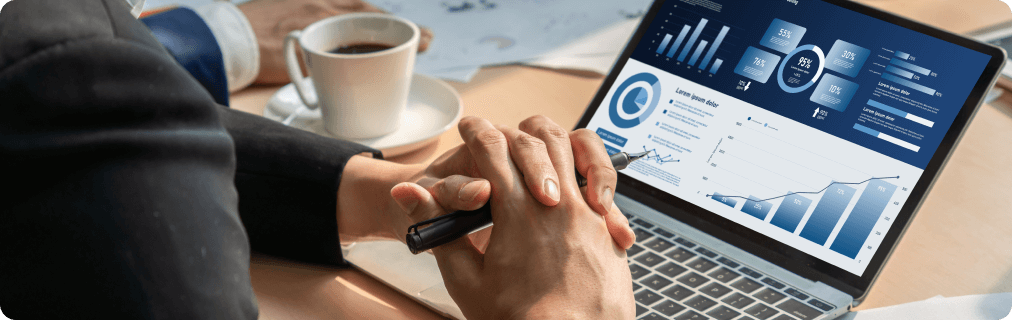
Algorithms and datasets to evaluate AI Agents of your choice