AI Terms for Beginners and Experts from A to Z
In this Artificial Intelligence glossary, we’ve gathered essential AI terms commonly used in tech and business by both AI industry beginners and experts.
It’s designed to deepen your understanding and help you navigate the AI systems by explaining industry-specific terms and showing how they connect to different AI solutions.
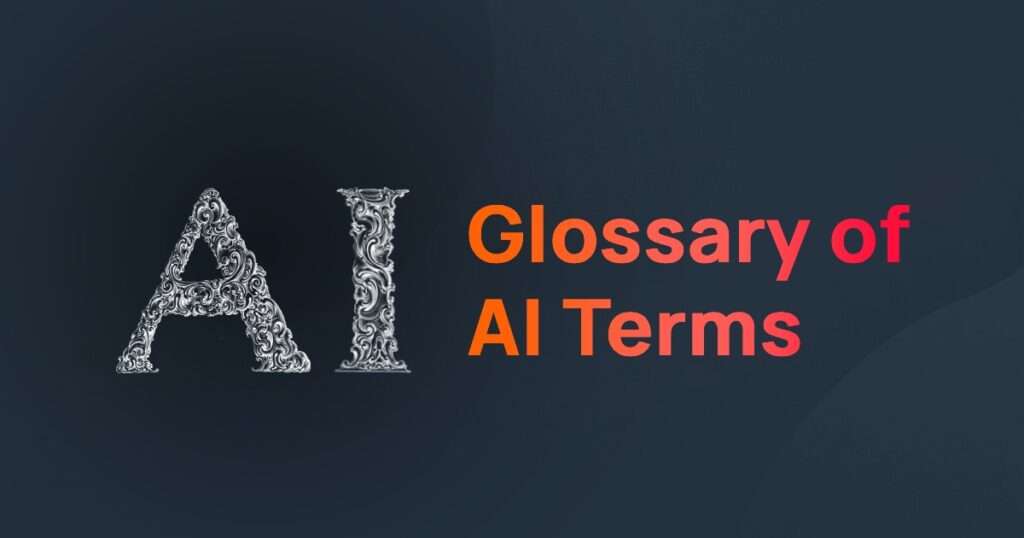
Artificial Intelligence (AI) Terminologies
Why Learning AI Terms is Important
In 1956, Dartmouth Professor John McCarthy organized a conference called “Cerebral Mechanisms in Behavior.” It was about computational learning theory and how computers could be used to think like the human brain. McCarthy also did something else that was very important at the conference: He coined the phrase “artificial intelligence.”
Since then, the field of Artificial Intelligence (AI) would go on to spawn many other AI terminologies, words, and acronyms. Many of them were technical or would just fade away. But of course, other AI concepts have become major categories. We gathered the most common terms in a glossary of AI terms for you.
Artificial Intelligence (AI):
Artificial Intelligence is the overarching term in this AI terms glossary. The field of data science focuses on developing intelligent machines capable of performing machine learning tasks that would typically require human intelligence but instead leverage machine intelligence powered by artificial intelligence technology.
AI Assist:
AI Assist is an AI-powered system that supports users by understanding queries, providing information, and performing tasks. It enhances productivity and convenience in various applications like customer service and virtual assistants.
AI Copilot:
An Enterprise AI Copilot is an AI-powered virtual assistant in an enterprise using advanced language models and generative AI, it offers human-like interactions, proactive notifications, and automated task resolution across multiple domains, including customer service, employee experience, and operational efficiency.
AI Discovery:
AI Discovery involves leveraging machine learning technology to extract valuable insights and patterns from large datasets. By using advanced algorithms and data-analysis techniques, businesses are able to uncover hidden correlations, trends, and anomalies, enabling data-driven decision-making and the discovery of new knowledge.
AI Ethics
AI ethics involves the moral principles and techniques intended to inform the development and responsible use of artificial intelligence technology, focusing on issues like privacy, bias, transparency, and the impact on society.
AI Observability:
AI Observability refers to the practice of monitoring, analyzing, and gaining insights into the behavior and performance of artificial intelligence systems. It involves collecting and analyzing structured data related to AI models, training processes, and deployments to ensure transparency, reliability, and effectiveness in AI operations.
AI Service Desk:
An AI Service Desk is an automated support system powered by artificial intelligence. It assists users in resolving their queries or issues by using natural language processing, machine learning algorithms, and other AI techniques, thereby improving efficiency and reducing response times.
AI Search:
AI Search also called Enterprise AI Search or AI Search for enterprise uses generative AI and large language models to understand natural language queries, offering personalized, relevant search results across various data repositories across the organization. It continuously learns from user interactions and organizational data, ensuring privacy and security, while providing intuitive, predictive search suggestions and real-time summaries.
AI Ticket Assist:
AI Ticket Assist refers to an artificial intelligence system used in customer service or IT service management. It automates the process of ticket creation, categorization, prioritization, and routing. It also assists in resolving tickets faster by providing relevant solutions based on past data and machine learning.
Virtual Assistants:
An AI-based software or application mostly called, AI Virtual Assistant, provides support, performs tasks, and answers questions through natural language interactions and conversational AI technologies, often utilizing speech and image recognition and synthesis.
Artificial Intelligence Operations (AIOps):
An AIOps platform leverages AI and machine learning techniques to IT operations that enable automated monitoring, detection, and resolution of issues or anomalies—transformative impact. AIOps stands for artificial intelligence for IT operations.
Augmented Intelligence
Augmented intelligence emphasizes AI’s role in enhancing human intelligence rather than replacing it. It focuses on AI’s assistive role, augmenting human abilities and intelligence with machine intelligence.
Bard:
An AI language model developed by OpenAI, specializing in generating creative written content, including poetry, stories, and scripts. It demonstrates a deep machine learning method based on models.
Big Data
Big data refers to extremely large datasets that may be analyzed computationally to reveal patterns, trends, and associations, especially relating to human behavior and interactions.
Chatbot:
A chatbot is a computer program or software application designed to conduct online text or voice conversations, simulating human interaction. Modern chatbots typically use AI technology, and their use cases are customer service, information retrieval, or entertainment.
ChatGPT:
ChatGPT is a Large Language Model (LLM), Also, an AI-powered conversational agent developed by OpenAI. It utilizes machine learning algorithms to generate human-like text based on user input, designed for engaging and coherent conversations.
Computer Speech Recognition:
The ability of a computer system to convert spoken language into written text, allows for machine translation of voice commands and dictation.
Conversational AI:
Conversational AI refers to the subfield of artificial intelligence that focuses on creating intelligent systems capable of interacting with machines and humans in human-like conversations.
Conversational AI Assist:
Conversational AI Assist refers to an artificial intelligence system designed to simulate human-like interactions. Through natural language processing and machine learning methods, it can understand and respond to user queries in a conversational manner, improving customer service experiences on digital platforms.
Conversational Automation:
Conversational Automation is a concept that combines the power of conversational AI and automation. It involves automating and streamlining interactions with users through chatbots or virtual assistants, enabling efficient and effective communication. By leveraging natural language understanding and automated processes, conversational automation enhances customer service, information retrieval, and task completion in a conversational manner.
Conversational Search:
A conversational Search is an approach to information retrieval that utilizes conversational AI techniques. Instead of traditional keyword-based queries, users can interact with search systems using natural language, similar to having a conversation. By understanding user intent and context, conversational search systems provide more relevant and personalized search results, improving the overall search experience.
Convolutional Neural Network (CNN):
CNN is a special kind of ANN designed to process data with a grid-like topology, like an image.
Data Annotation:
Data Annotation is the process of labeling data, including images, videos, text, and audio, with specific annotations or tags. This task, carried out by data annotators, involves identifying key information within the data and marking it with appropriate labels, enabling AI systems to interpret the data accurately.
Data Mining:
The process of discovering patterns in raw data and extracting valuable insights from large datasets using AI and machine learning models.
Deep Learning Models:
A subfield of machine learning that utilizes artificial neural networks with multiple layers to process and analyze complex patterns and structured data, mimicking the human brain’s structure and function.
DevOps:
The software development approach emphasizes collaboration, communication, and automation between software developers and IT operations teams to deliver applications more rapidly and efficiently.
Domain Specific LLM
Domain-specific LLM is a language model that has been tailored for specialized fields, addressing the broader and less focused nature of Generic LLMs, which are trained across multiple domains.
Edge Computing:
A computing paradigm that brings AI and data processing closer to the source of data generation, reducing latency and enabling real-time analysis and decision-making on devices or at the network edge.
Enterprise Search:
Enterprise search refers to a sophisticated system designed for efficiently locating and accessing organizational information. It comprehends a range of data types and supports real-time, dynamic response generation for complex queries using advanced retrieval technologies.
GPT:
GPT, or Generative Pre-trained Transformer, is a state-of-the-art language model that uses transformer architecture and pre-training techniques to generate human-like text based on a given input.
GPT-3:
GPT-3, developed by OpenAI, is a state-of-the-art language processing AI model. It generates human-like text by predicting subsequent words in a given piece of text. It’s versatile and used for tasks like translation, answering questions, and creative writing.
GPT-4:
GPT-4 is the latest version of GPT as of June 2023, and a large language model created by OpenAI that can generate text, translate languages, write different kinds of creative content, and answer your questions in an informative way.
Generative Adversarial Networks (GANs):
A type of AI model that involves two neural networks—the generator and the discriminator—competing against each other, resulting in the generation of realistic data or images.
Generative AI:
Generative AI refers to a subset of artificial intelligence that focuses on creating new and original content, such as images, music, or text, using algorithms and machine learning models. It enables machines to generate creative outputs that mimic human-like patterns and styles.
AI Hallucination:
AI hallucination typically results from overfitting, bias, or a lack of context awareness in the machine learning model. The technologies like an image generator or large language model, that generate outputs that are not directly derived from the input data can hallucinate if not trained not to do so.
Large Language Models (LLMs):
Large Language Models are AI models that generate human-like text based on a given prompt or context, aiming to capture the statistical properties and patterns of natural language. If you choose Aisera for LLM as a Service it helps you to buy, bring, or build your LLM.
LLM Evaluation:
LLM evaluation is a detailed process used to assess the performance and abilities of large language models, revealing their strengths and weaknesses. This helps developers refine these models and choose the ones that best meet project needs. It begins with a concise overview of LLMs.
LLM Gateway
The LLM Gateway serves as a vital intermediary for the Large Language Model (LLM) service, expertly managing user requests and responses. It plays a key role in post-processing, significantly enhancing the effectiveness and safety of LLM interactions. This gateway adds substantial value and functionality to the output of the LLM service.
LLMOps
LLMOps is an emerging field in AI, that focuses on the deployment and management of advanced language models, integrating technology and strategy to adapt machine learning operations for large-scale language applications.
Machine Learning System (ML):
Machine learning is a subset of AI that involves the use of algorithms to enable computer systems to automatically learn with reinforcement learning and pattern recognition from data and improve their performance without being explicitly programmed.
Artificial Neural Networks:
Mathematical models inspired by the human brain’s interconnected network of neurons. Neural networks are used in AI and machine learning models to recognize patterns, make predictions, and classify data.
Neural Search:
Neural Search is an advanced search technique that harnesses the power of machine learning technology, particularly deep learning algorithms, within neural networks. By utilizing these sophisticated algorithms, neural search systems can process complex search queries, enabling more precise and contextually relevant search results. This approach optimizes search engines to enhance the user experience by using the capability of machine learning and deep learning.
Next-Gen IT Service Management (Next-Gen ITSM):
Next-Gen IT Service Management refers to innovative strategies in managing IT services, typically involving automation and artificial intelligence. This could include AI-powered service desks, predictive analytics, cloud services, and other advanced technologies aimed at enhancing service delivery and operational efficiency.
Natural Language Generation (NLG):
The process of generating human-like language or text using AI algorithms, allows machines to produce coherent and meaningful written content.
Natural Language Processing (NLP):
Natural language processing in AI is the ability of a computer system to understand, interpret, and generate human language, enabling interactions between humans and machines through speech or text.
Natural Language Understanding (NLU):
The AI’s capability to comprehend and interpret human language in a meaningful way, allows machines to extract intent, context, and relevant information from textual or spoken inputs.
Omnichannel AI Support:
Omnichannel AI Support refers to an artificial intelligence system that provides customer service across multiple communication channels, such as email, social media, phone calls, and live chat. It ensures seamless, consistent, and personalized customer experiences, regardless of the platform used for interaction.
Predictive Analytics:
The use of statistical models and AI algorithms to analyze historical data and make predictions or forecasts about future events or outcomes.
Robotic Process Automation (RPA)
Robotic process automation (RPA) is a type of business operation enhancement utilizing software robots (bots) or AI-driven tools. It’s well known for its role in Conversational Automation, or workflow Automation. Sometimes, it’s termed software robotics, distinct from robot software.
Sentiment Analysis:
A technique that uses AI to analyze text or speech and determine the customer sentiments or emotional tone expressed, such as positive, negative, or neutral.
Supervised Learning & Training Data:
In Supervised Machine Learning, an AI model learns from labeled training data. For instance, if you wanted the model to recognize cats, you’d train it with thousands of images of cats as training data, each explicitly labeled as a cat.
Unsupervised Learning:
With Unsupervised Machine Learning, the model learns from unlabelled data. Instead of telling the model what to look for, you let it figure things out on its own. It’s a bit like throwing a kid into a pool and letting them learn to swim.
Universal Bot:
A Universal Bot is an advanced AI-powered chatbot designed to interact across multiple channels and platforms. It’s capable of understanding and responding to user queries in a consistent, coherent manner, providing a unified and seamless customer experience regardless of the communication medium used.
Workflow Automation:
Workflow Automation, incorporating machine learning, is the process of using software or tools to automate manual tasks or workflows in a business environment. This advanced form of automation learns and improves over time, reducing human intervention, minimizing errors, and enhancing efficiency and productivity.
Final Thoughts on learning AI Terminology
Understanding common AI terms is essential for harnessing the power of artificial intelligence in your business operations. By familiarizing yourself with concepts like machine learning, deep learning, natural language processing, and neural networks, you can explore the potential of AI solutions to drive innovation, efficiency, and growth.
Our blog provides valuable insights into how businesses can leverage AI technologies to gain a competitive edge and achieve their goals. Dive into our articles to discover practical ways to utilize AI in your organization and unlock its transformative impact. We consistently update this list to encompass new terms and their definitions.