AI-native should be viewed not just as a technology strategy but as a fundamental redesign of organizational systems with AI systems at their core. The pace of artificial intelligence (AI) advancement is not just continuing; it’s accelerating, transforming every corner of the industrial and technological landscapes.
Generative AI, in particular, has become a cornerstone of this revolution, enabling the creation of new content—from textual articles to complex video simulations—based purely on initial input and contextual data. This capability is redefining potential across various sectors, from healthcare to entertainment, offering new business opportunities and driving innovation.
AI-native companies are at the forefront of this transformation. Unlike traditional businesses, these organizations are architected from the ground up to integrate and capitalize on advanced AI technologies without the encumbrances of outdated systems. This foundational AI integration allows them to swiftly adopt technological advancements, positioning them as leaders in innovation and adaptability.
The transformational power of AI is vividly evident across the telecom industry. AI native applications leverage a data-driven, knowledge-based ecosystem; they are rapidly replacing static, rule-based systems with the ability to learn and adapt when needed. Information is consumed and dynamically generated to realize new AI-based functionalities or augment and increasingly replace static mechanisms.
Just what is AI-Native Networking, and how is it rescuing the telecom domain from the consequences of its progress? Because only AI-Native solutions can enable enterprises to meet the insatiable needs confronting advanced intelligent telecom networks. Let’s define AI-Native networking, explore how it fits into telecommunications, discuss the benefits it delivers, and calculate the future directions it is taking.
The Evolution and Impact of AI-Native Systems
Simply put, AI-native refers to systems and companies fundamentally designed with AI at their core; it means that AI is engineered intrinsically from the ground up and is a pervasive core component of operations and services.
This is profoundly different from merely integrating AI into existing systems by replacing or retrofitting an existing technology component with one that enables AI capability. AI is far too revolutionary to be merely “bolted on.” The challenges now arising call for the ability to fully exploit AI throughout every entity of the organization, end to end. AI-native systems can significantly reduce network trouble tickets by proactively identifying and resolving issues before they disrupt the user experience.
The Emergence of the AI Native
Today, we comprehend Native AI as a congenital, inseparable, and organic part of a system—as natural to its function as if born in a living organism. Over the last decade, AI has evolved from an exotic and mind-bending mythology to a pragmatic element of working technologies. Machine Learning (ML) enables heretofore impossible feats and functions as AI capabilities mature at amazing rates. We are now mastering the means to accurately capture patterns and correlations in available data—however complex that input is.
AI native products, designed from the ground up with artificial intelligence at their core, enhance efficiency and innovation within their respective fields. Companies like Jasper and Midjourney exemplify this approach, showcasing how AI-native products can revolutionize industries.
In the telecom domain, AI use cases prove how AI can be adopted and become a stepping stone for the growth and improvement of network performance. AI-based solutions enable increasing intelligence and self-learning in network automation.
It’s a small wonder that AI is becoming an increasingly pervasive, eagerly awaited, and quickly implemented proof of telecom progress. An AI-native implementation supports a data-driven, knowledge-based ecosystem. That data and knowledge are absorbed and comprehended to help bring to life new AI-based functionalities. At the same time, AI is replacing static, manual, and rule-based activities with learning and adaptation.
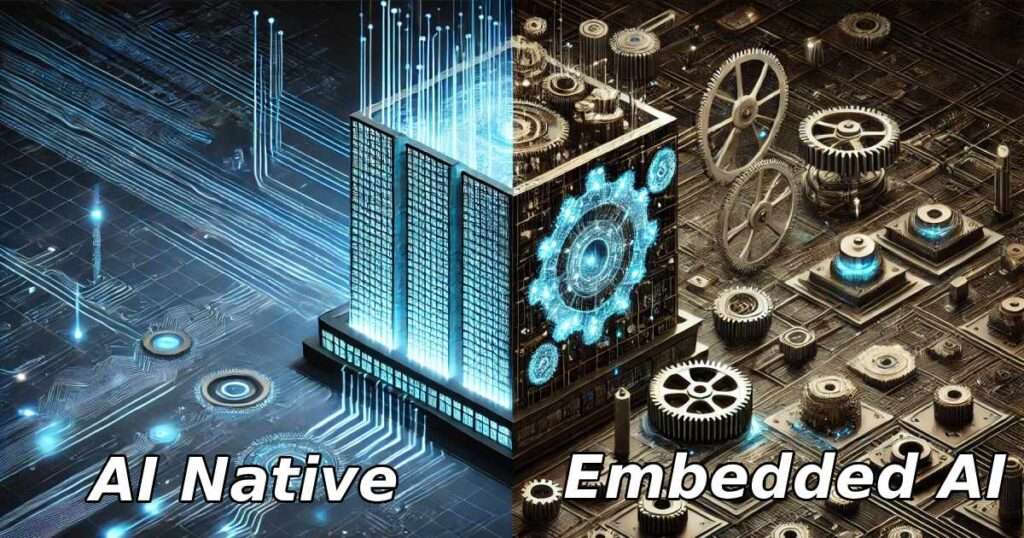
AI Native vs. Embedded AI: Understanding the Distinctions
AI-native and embedded AI represent two distinct approaches to integrating artificial intelligence within technological frameworks, each with its unique implications for business operations and technological evolution.
AI Native: A Foundation Built on AI
AI-native technology is designed from the ground up with AI at its core. This approach doesn’t just integrate AI; it centers the entire system around AI functionalities. The primary advantage of AI-native systems is their inherent capability to seamlessly incorporate advancements in AI technology. These systems are built to leverage AI to its fullest potential, enabling rapid adaptation to new innovations and changes in the market. By doing so, AI-native architectures can drive transformational changes across industries, enhancing efficiency and enabling new business models that were previously unfeasible.
Embedded AI: Enhancing Existing Systems
On the other hand, embedded AI involves the integration of AI functionalities into pre-existing technology systems. The goal here is to enhance and improve the performance of these existing systems. Embedded AI can be implemented in several ways:
- Component Replacement: This method involves replacing a current technology component within the system with a new one that incorporates AI capabilities, thereby enhancing the system’s functionality.
- Addition of AI Components: Another common strategy is to add AI-based components to an existing technology stack. Whether these components operate independently or as part of an integrated API interacting with external AI services, they are designed to offer backward compatibility, ensuring that they work seamlessly with the old systems without requiring extensive modifications.
- Legacy System Optimization: A particularly challenging application of embedded AI is its use to control and optimize legacy systems. In this scenario, AI components are specifically engineered to interface with outdated technologies. This approach allows organizations to breathe new life into their legacy systems, enhancing their efficiency and extending their operational life without the need for complete system overhauls.
Strategic Implications
Choosing between AI-native and embedded AI depends largely on the specific needs, strategic goals, and existing technological infrastructure of a business. AI-native solutions are ideal for new projects or systems where AI is intended to be a central feature from the outset. In contrast, embedded AI is suited for upgrading existing systems, allowing businesses to gain AI benefits without discarding their current investments.
Both approaches offer significant benefits, but the decision to opt for AI-native or embedded AI should be informed by a thorough analysis of the organization’s long-term technological and business objectives. As industries continue to evolve with advancements in AI, understanding these distinctions becomes crucial in positioning businesses at the forefront of innovation and competitive advantage.
AI Native Solutions Across Industries
AI-native frameworks are adeptly leveraging generative AI to revolutionize various industries, propelling a wave of innovation and strategic advancements. By embedding these sophisticated technologies into their core operations, companies are unlocking new realms of efficiency and creativity, paving the way for transformative applications that range from personalized healthcare treatments to dynamic retail experiences and beyond.
This integration of AI-native and generative AI technologies is reshaping industries by enhancing their ability to adapt, predict, and efficiently meet the evolving demands of their markets.
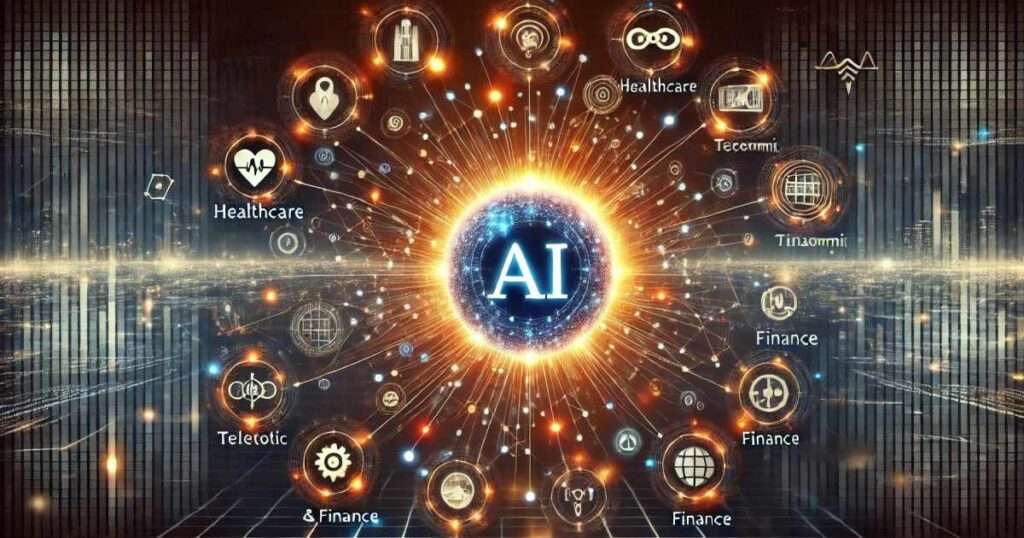
AI Native in Banking: Leveraging GenAI for Enhanced Financial Services
The adoption of Large Language Models (LLMs) and Generative AI in banking represents a pivotal shift towards more personalized, efficient, and secure financial services. AI-native strategies in banking are profoundly leveraging generative AI to pioneer transformative changes, particularly through the use of Large Language Models (LLMs).
This integration marks a significant evolution in how banks operate, enabling them to deliver more personalized, efficient, and secure financial services. By embedding AI at the core of their operations, banks can harness these powerful models to enhance various aspects of their services, from customer interaction to backend processing.
This approach not only streamlines operations but also significantly improves the accuracy and customization of financial services, ensuring that clients receive solutions that are tailored specifically to their needs and preferences. Banks utilize generative AI to:
- Enhance Customer Interaction: AI-driven chatbots and AI assistants, powered by LLMs, handle customer queries and transactions with unprecedented speed and personalization.
- Improve Fraud Detection: Advanced ML models analyze transaction patterns in real time to identify and prevent potential fraud.
- Optimize Loan Processing: AI systems streamline the loan approval process by quickly assessing credit risk based on an array of customer data points.
- Financial Forecasting: Generative AI models predict market trends and customer behavior, aiding in more accurate financial planning and risk management.
AI-Native Strategies in Healthcare
AI-native strategies in healthcare are revolutionizing the industry by leveraging generative AI to enhance diagnostic accuracy and personalize patient care. By integrating these advanced technologies, healthcare providers are transforming how they deliver services, achieving greater efficiency and precision. Here’s how AI-native systems are making a significant impact:
- Improved Diagnostics with AI: Generative AI models are transforming the interpretation of medical imaging data, allowing for earlier and more accurate detection of complex conditions. This technology significantly enhances the capability of healthcare systems to diagnose diseases with a level of precision previously unattainable.
- Personalized Treatment Plans: Leveraging the power of generative AI, healthcare providers can develop treatment plans tailored to individual genetic profiles. This personalized approach ensures treatments are more effective and side-effect profiles are minimized, suited specifically to each patient’s unique biological framework.
The deep integration of generative AI in healthcare operations not only streamlines processes but also continuously improves them by learning from expansive datasets of genetic and medical information. This ongoing adaptation enhances the capability of healthcare systems to refine patient care strategies, leading to:
- Enhanced Patient Outcomes: The ability of AI-native systems to adapt and optimize treatments over time improves overall patient outcomes, offering therapies that are increasingly effective and responsive to individual health needs.
- Operational Efficiencies: AI-native healthcare systems improve operational efficiency, reducing the time and resources spent on diagnostics and treatment planning, and allowing healthcare professionals to focus more on patient care rather than administrative tasks.
These advancements exemplify the transformative potential of AI-native structures in healthcare, setting new standards for medical treatment and management that prioritize responsiveness, personalization, and effectiveness.
Understanding AI Native in Telecom
AI native in telecom refers to platforms designed from the ground up with AI at their core. These platforms are optimized for AI functionalities, providing advanced solutions such as AIOps, which utilize data from user experiences to:
- Reduce Problems: By proactively identifying issues before they escalate, minimizing disruptions.
- Intelligent Ticketing: Automating the process of creating and managing support tickets.
- Incident Resolution: Handling incidents without requiring constant human intervention, thus enhancing efficiency and reducing response times.
- Network Trouble Tickets: AI-native systems can significantly reduce network trouble tickets by proactively identifying and resolving issues before they disrupt user experience.
An AI-native solution also involves the development of standalone AI-based services and their integration into existing platforms. For example, Google’s acquisition of DeepMind shows how companies can innovate by either creating or acquiring AI-native solutions to enhance their offerings.
Purpose and Environment of AI Native Implementation
The purpose of AI native implementation is to solve complex problems that challenge even deep domain expertise. This involves:
- Zero-Touch Networking: Autonomous AI native systems identifying anomalies and providing automatic remediation through advanced machine learning and robust correlation.
- Environmental Awareness: AI-native systems detect and adapt to environmental conditions, such as database types, hardware platforms, and radio domains, to optimize performance.
Intelligence, System, and Outcome
AI-native systems are characterized by their ability to:
- Integrate Base Knowledge with New Observations: Continuously adapt to changing circumstances and expand their knowledge base.
- Create Strategic Courses of Action: Develop strategies to achieve goals by absorbing and utilizing new information.
These systems manage the lifecycle of AI-based functionalities, ensuring:
- Trust and Fairness: Operations are transparent, explainable, and trustworthy.
- AI Safety and Control: Implementing safety mechanisms and sustaining collaborative intelligence across the network.
The outcome of AI-native implementations includes:
- Cognitive and Autonomous Network Vision: Enabling networks to reason and make decisions based on available knowledge.
- Value-Added Functions: Enhancing network architectures with AI-centric capabilities, improving overall efficiency and effectiveness.
By embedding AI deeply into telecom operations, companies can create adaptive, intelligent ecosystems that respond dynamically to market changes and consumer demands. This integration leads to more resilient, efficient, and high-performing networks that meet the evolving needs of the digital age.
AI Native Architecture and Maturity of AI Models
The AI native maturity model illustrates a baseline assessment of where a product is located in its AI native journey, from concept to full implementation. Maturity measures whether the architecture delivers the desired capabilities in the right combination to satisfy customers, shareholders, and employees.
This representation can help measure the degree, and situate which stage of “AI nativeness” a product or system has reached. It also helps set a target and milestones to fully manage AI, advancing from practice to performance, as Accenture sees it.
AI Native Solutions and Use Cases
An AI-Native Networking Platform has two major use cases. When it comes to AI for networking, AI is vital for IT operations (AIOps) to identify patterns, predict network behavior, and detect anomalies. It creates proactive evaluations and automated corrections to optimize user experiences.
Integrating AI solutions into products and services can significantly enhance functionality and user experience, leveraging brand recognition to introduce innovative features.
Also prominent is networking for AI, a huge market that serves the data center networks that are so important for AI training and workloads. These landscapes require every GPU in the system to smoothly connect and communicate, for example. Also vital is low power consumption and agility. These all work together and make the network critical to the success of AI implementation.
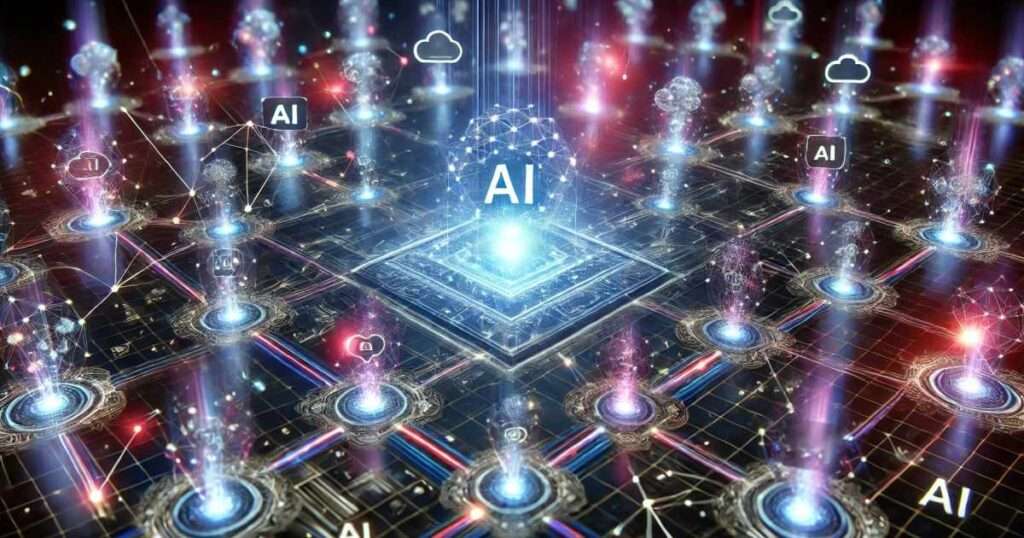
Exploring AI-Native Networking
Once an organization puts an AI-native networking solution in place, they can proactively discover the details of network performance issues long before they damage the user experience—a real game changer for IT and network operations. Organizations can transition from reactive to preemptive troubleshooting, predicting—often in real time—what is needed to manage all of this responsibility. Success improves the lives of end users, customers, and the general public.
By leveraging AI, AI-native networking solutions fully utilize artificial intelligence to enhance operational efficiency, creating systems where AI is integral to their functionality and decision-making processes. AI systems enhance network operations by continuously validating operations against specified intents.
The profound impact on these areas is how AI-Native Networking transforms global digital connectivity. It extends AIOps capabilities across all facets of network operations. For instance, leveraging AIOps for cloud systems, encompassing everything from end-user devices to massive application infrastructures, AI-Native Networking is revolutionizing persistent challenges in maintaining efficient, reliable network performance—mitigating network outages, expediting troubleshooting, preemptively solving problems, and minimizing application downtime.
The Importance of AI-Native Networking
In fact, the benefits of AI-Native Networking are making possible unprecedented levels of automation, intelligence, and reliability. By leveraging AI algorithms to automate tasks and analyze vast volumes of data predicting network behavior, AI-Native Networking promises to keep elevating the current state of network connectivity. AI technology improves network functionality and accessibility, enabling companies to gain a competitive edge. Organizations can unlock new business potential and drive network evolution into awesome levels of innovation, agility, and efficiency.
Enhanced Efficiency and Performance
The value of efficient network performance cannot be overstated. AI algorithms work to improve network traffic routes, allocate bandwidth optimally, and minimize latency. The algorithms conquer persistent headaches that have held back network performance and efficiency from the start, enabling unprecedented speed and dependability. Bandwidth-intensive applications that benefit the most include video streaming, high-scale cloud computing, and the enablement of effective AI training and inference capabilities.
Predictive Maintenance and Downtime Reduction
The value of preemptive surveillance, detection, and resolution of issues is another major benefit of AI-native networking. Keeping seamless, intensive observation and proactively taking action, AI-native networks can schedule needed maintenance, reduce or eliminate unexpected downtime, and repair issues before they affect end users and their projects. Network availability is crucial to competitiveness, operations, revenue, and brand reputation.
Improved Security
AI-native networks bring the capability to handle massive volumes of network data in real-time. Unlike human-driven scrutiny and vigilance, AI-native networks are on duty 24/7 to track potential anomalies and cyber threats. Although cybercrime is a constantly moving target, AI-native networks are capable of keeping pace with security threats and getting ahead of campaigns to warn targets and protect sensitive and confidential data.
Cost Savings
Manual observation and intervention are costly and not fail-safe. Automation of network management substitutes seamless efficiency, plus the tireless functionality of AI, paving the route to significant cost savings in labor and operations. Of course, predictive maintenance obviates costly downtime and emergency repairs—a relief for the company, customers, and employees.
Scalability and Flexibility
Every manual activity associated with the network opens a potential for inaccuracy and error. Manual AI-native networks, however, are built from the ground up to take on and implement change without the need for manual reconfiguration. This major relief and reassurance allows the network to deal with scaling workloads and incorporate the latest innovative devices seamlessly and effortlessly.
Enhanced User Experiences
Users benefit in countless ways from AI-native networks. Not only is their work product optimized, but their attention can be confidently focused on higher-value tasks. The user experience has been proven over and over to benefit substantially, ensuring that IT operators, employees, consumers, and users of public internet services are satisfied and remain loyal.
How AI-Native Networking Works
The highest quality, most accurate data is vital to AI-native networking. If you want your AI-native network to deliver the most value, it needs the ability to absorb and process not only huge data volumes but data of unquestionable quality and reliability.
The consequences of bad or unreliable data are as difficult to bring under control as a forest fire—and just as destructive: inaccurate or biased responses, wrong answers; and damage that manifests organization-wide. Think of all the aspects that the network relies on—traffic patterns, device performance metrics, network usage statistics, security logs, real-time wireless user states, and streaming telemetry from routers, switches, and firewalls. Then think of how wrong they can go under manual control!
Natural language processing (NLP) plays a crucial role in enhancing communication between humans and machines, enabling applications such as voice interactions, sentiment analysis, and language translation.
It’s up to the machine learning algorithms to analyze collected data. Algorithms must, of course, be trained to recognize data patterns and anomalies. They learn from the network’s behavior over time, sharpen their accuracy, and become ever more reliable. The benefits arrive when the predictions and decisions they make are on target and serve as guideposts for successful strategic decision-making and investing.
Today, users can feel increasingly confident about trusting results yielded by a system’s ML algorithms. The insights are key to understanding how data is being utilized. The network can provide the optimal response in real time; working dynamically, it can adapt smoothly and quickly to changing conditions and deliver results, in such areas as:
Predictive modeling: AI can deliver predictions on network states or issues such as forecasting traffic spikes or warning about weak spots in the network vulnerable to failure or attack.
Self-optimization: AI-native networks not only detect upcoming new problems, they predict those problems based on past behavior. For example, AI can see warning signs of bottlenecks at certain times of day or spikes that can slow traffic and impact network performance. They preemptively reroute traffic to sustain network performance—and the trust and satisfaction of users.
Proactive maintenance and self-healing: It’s up to the network to detect and diagnose issues preemptively—before they explode into significant problems like hardware failures. Even more amazing is the ability to automatically correct the anomaly, whether as simple an act as rebooting a device—or elevating to human intervention for the most challenging, abstract issues.
Security enhancement: Security lapses have the potential to cause greater loss of revenue, brand, Intellectual property, customers, and employees than any other challenge. Think of the value of a network’s ability to detect a threat, implement security protocols, isolate affected or threatened network segments, or neutralize malicious traffic.
User experience management: AI-native networking, with its ability to customize and personalize activities and performance to meet demands, is a user hero. Supporting users by helping them sequence priorities and preferences while optimizing their use of resources (such as time) has remarkable effects on user behavior, retention, and morale.
Aisera as an AI Native Company
As an AI pioneer and innovator, Aisera built the landmark GenAI and AiseraGPT on a native enterprise LLM from the ground up. The company has earned industry-wide respect for its ability to fast-track the digital transformation journey. User and service behavioral intelligence drives end-to-end automation of tasks, actions, and business processes, delivering on the promise of AI for a growing span of AI customer service, including Adobe, Chegg, GAP, Grant Thornton, and others.
As Forrester says in the Forrester Wave™ Leader 2024, “The strength of Aisera’s current offering comes from its variety of functionality, with strong support across agent augmentation, automation facilities, prebuilt assets, and automated workflow development… ideal for customers wanting both a configurable platform, as well as those who need a vendor that will work to help them succeed…”
Aisera’s AIOps Platform predicts, detects, and resolves anomalies, major incidents, and performance issues, even before they impact performance. The platform analyzes incidents from ticketing and telemetry data (MELT); from infrastructure, apps, and services to identify root causes, all while reducing time to detection and resolution.
With its unified interface, Aisera’s Enterprise AI Copilot provides proactive support across multiple domains, channels, languages, and bots. Users no longer need to interact with each department’s separate AI Copilot. Rather, AI Copilot uses the power of your relevant third-party copilots (Microsoft Copilot, Salesforce Einstein, and more) to execute their services. This allows users to deliver proactive assistance, no matter the domain.
Aisera’s advances in AiseraGPT and Generative AI enable agents to inquire and receive proactive notifications about infrastructure health status, issues, and root causes, using natural language. That means Aisera’s AI Copilot for AIOps converts cryptic technical alerts—usually requiring an expert to decipher and interpret—into easily human-readable notifications.
Aisera streamlines ongoing management challenges while assuring that every connection is reliable, measurable, and secure. They are building highly performant and adaptive network infrastructures that are optimized for the connectivity, data volume, and speed requirements of mission-critical AI workloads. Aisera’s AI Copilot is built upon the TRAPS Framework, which aims to accelerate the time-to-value of Generative AI while addressing and minimizing potential risks. With this framework, Aisera delivers an enterprise AI Copilot that is trusted, responsible, auditable, private, and secure.
Conclusion: The Future of AI-Native
The future of AI is being shaped by the AI-native concept, which embeds AI as a foundational element within organizational infrastructures to enhance decision-making and customer interactions. Aisera is pioneering this future with its advanced Generative AI technologies, which are pivotal in both AI-native and embedded AI environments.
By integrating these innovative solutions, Aisera ensures that businesses are equipped to adapt and scale their AI strategies efficiently. This approach not only addresses current needs but also sets a robust foundation for the future, empowering organizations to leverage the transformative potential of AI and secure a competitive advantage in a rapidly evolving digital landscape. Book a free custom AI demo for your enterprise to experience the power of Aisera’s GenAI in action!