An Introduction to the Concept of Generative AI and Predictive AI
The words that define AI “generative” and “predictive,” represent complex and deeply meaningful concepts. Why is it so important to differentiate them? What do they tell us, and what unique functions do they carry out for us? This blog is going to attempt to untangle and define the terms generative and predictive; to demarcate the differences between these key, multi-syllabic words, and dive into their usage.
For decades, AI has been emerging from its origins of hard science, engineering, and mathematics into the “real” world that we humans occupy. The words generative and predictive are foundational to that process. How did they gain their names, and why is it so important to discern their contrasts?
Generative models create new, realistic content by learning from existing data patterns.
Understanding Predictive AI
Predictive AI works by merging statistical analysis with machine learning algorithms to discover data patterns and forecast future outcomes. The technology extracts insights from historical data to make accurate predictions about the likeliest emerging event, result, or trend.
Predictive AI models rev up the speed and precision of the predictive analytics which are usually employed for business forecasting for example, to project sales, estimate demand for a service or product, personalize customer experiences, and optimize logistics. Thus, predictive AI helps enterprises make accurate, informed decisions on the smartest next step to take.
Definition and Core Concepts
Predictive AI (also called predictive analytics) is a subset of AI that uses historical data and machine learning algorithms to analyze patterns and predict future events or trends. As mentioned above, the goal of predictive AI is to help companies and individuals make accurate, sharp, wise decisions by revealing likely outcomes based on what has gone before.
How Predictive AI Models Work
Predictive AI trains machine learning algorithms on this historical data to expose patterns, relationships, and trends. Based on the training data, these models extract insights to make predictions about future occurrences. Humans alone would likely miss these key insights or relationships; however brilliant a person or team of people, we don’t have the robust calculating capabilities of a powerful computer to visualize these insights,
For example, predictive AI is a perfect fit for certain industries, in particular finance and marketing, to forecast customer behavior, stock market trends, or product demand. By analyzing vast datasets and applying complex algorithms, predictive AI reveals astonishingly accurate insights and sharpens the process of decision-making.
Understanding Generative AI
Generative AI trains models to create original (or “fake”) content. These models learn from masses of existing data available on the internet and other visible places. It then generates “new” data similar but not identical to the original, based on those patterns. Generative AI tools offer outputs that are not copied or plagiarized exactly from the training data but rather are inspired by it. GenAI responds to a prompt or request with this generated “original” content, such as text, audio, molecules, natural images, software code grammar, text, or video.
There is no shortage of raw data for generative AI models to train on; we humans pour out avalanches of data. Models draw from encoded patterns and relationships in this training data to understand user requests and create relevant new content similar but not identical, to the original data source.
Most generative AI models start with a foundational deep learning model that yields statistically probable outputs after being prompted. Large language models (LLMs) are the source for generating text, but diverse foundation models are only a click away for different types of content generation.
4 Key Differences Between Generative and Predictive AI
Here is what to know when differentiating these two forms of AI: Generative AI and predictive AI are each in a class by themselves, with predictive AI focused on solving problems, while GenAI works to create something imaginary. Predictive AI’s ability to forecast trends supports businesses in making informed decisions, while generative AI addresses issues in more complex domains using more complex mechanisms.
Let’s examine the two technologies and the key differences more deeply. Predictive AI has its own terrain, and we guard its boundaries vigilantly. It’s an awe-inspiring tool that opens up new spaces and abilities for business in particular. Generative AI is the mystical genii that responds to a rubbing of the lamp with original content audio, images, software code, text video, and seemingly infinite other formats. Where does it find such diversity? It embarks on an ML-powered journey through massive volumes of raw data, one of its most controversial powers.
1- Purpose and Goals
We’ve made the point that GenAI primarily focuses on creating new content, such as images, videos, music, or text. The goal we have created for it is to generate novel, creative outputs that mimic human-like patterns. In contrast, predictive AI analyzes archival patterns it perceives in data to forecast potential outcomes or trends. As its name implies, its purpose is to draw conclusions about future events and give the user a competitive business or financial advantage of some type. Of course, competitors are using their own systems to achieve victory in their own marketplace or stock market battles, so we can assume that an AI tool does not necessarily predict the winner of a struggle.
2- Input and Output Requirements
GenAI requires an initial input a prompt, a seed, and an example to ignite the creative process. It then spews out novel new content at a terrific speed based on this input. Contrarily, predictive AI relies on statistical formulas based on historical training data as input to make its forecast. The output of generative AI is innovative content that may be entirely new or may rely on previous has probably never existed before, while predictive AI peers into the future and reads it to come up with useful predictions.
3- Training Data and Model Architectures
GenAI systems use various technologies like neural networks, generative adversarial networks (GANs), and reinforcement learning to digest patterns from this training data and produce creative new outputs.
As discussed above, predictive AI leans on statistical algorithms and machine learning models to analyze data and identify patterns that it offers to predict future outcomes. The training data for generative AI consists of examples of the content type it should create, while predictive AI looks to the “real” past for historical data related to the specific event or outcome we wish it to predict.
4- Explainability and Interpretability
In many ways, AI is as incomprehensible as maps of the world drawn during the Middle Ages. The AI topography of GenAI models is a dark mystery; we say it “lacks explainability.” That could be interpreted as jumping out ahead of human comprehension, leaving us puzzling to decode the results we see, and the decision-making processes that yielded them up. Predictive AI speaks to our need for control because it’s grounded on numbers and statistics. But those can be inaccurate. As mentioned earlier, interpreting these estimates correctly still relies on human judgment. An incorrect interpretation can still lead a business to a wrong turn.
Key Differences | Generative AI | Predictive AI |
Purpose and Goals | Creates new content (e.g., images, text) to mimic human patterns. | Forecasts future outcomes to provide business insights. |
Input and Output Requirements | Needs a prompt or example to generate new content. | Uses historical data to make predictions. |
Training Data and Model Architectures | Uses neural networks, GANs, and reinforcement learning. | Uses statistical algorithms and machine learning models. |
Explainability and Interpretability | Often lacks clear decision-making transparency. | Relies on statistical data; requires human interpretation. |
Why Understanding the Gen AI and Predictive AI Differences Matters
You can use a metaphor of offspring to differentiate predictive and generative AI they are the children of AI. Imagine machine learning as their teacher, instructing powerful computers with great potential to learn from examples and make choices. Such computers can make predictions without being told exactly what to do. Think of ML as teaching computers to learn from examples and make their own decisions.
It’s an element of AI and LLM strategy that enables computers to predict things or make choices without being told exactly what to do.
You can’t imagine predictive AI without machine learning. Predictive AI uses ML techniques to build predictive models. What are these, we ask? They are engines that analyze historical data, pick up on patterns, and use them to forecast future events or outcomes. Years ago, we could only dream of computers powerful or sophisticated enough to model the weather or stock prices. Those concepts were beyond our unsupported capacity.
Generative AI algorithms, on the other hand, learn by analyzing and deriving patterns from input data. This input data is crucial for the model’s ability to create new outputs that resemble the original.
Human-driven stock pricing in the past relied on guesses, flukes, or (all too often) fraudulent manipulation. Weather forecasts were frequently punch lines. Not funny, however, was the potential for unanticipated disease outbreaks originating in obscure geographies or linked to human activities. The disease itself is a product of evolution, which has shaped life since the first cell division. Businesses failed or succeeded due to luck and global issues like war or revolution. In other words, happenstance. We don’t rely on solar eclipses anymore to predict our fortunes but are still struggling to achieve reliable forecasts.
Why Understanding the Gen AI and Predictive AI Differences Matters
At present, most generative AI models are said to “lack explainability.” People are puzzled by the decision-making processes that take place to yield their results. On the other hand, predictive AI estimates owe their accuracy to being grounded on hard numbers and statistics. Nevertheless, human judgment is still at the core. The turbulent state of world affairs today only proves how incorrect or irresponsible human choices and behavior create an unsound environment.
When people use generative AI (GenAI) to craft a new image or use ChatGPT, they are using AI but not ML anymore; rather, they rely on another subset of ML called deep learning neural networks of many layers modeled after the human brain.
They are exceptionally powerful in extracting intricate conclusions from large, diverse datasets. When we visualize something, our brains process the image in a way similar to how neural networks process input, mimicking the human brain (though the brain is far more complex than any neural network at least so far). GenAI uses this complexity to create fake concepts, including misleading AI hallucinations.
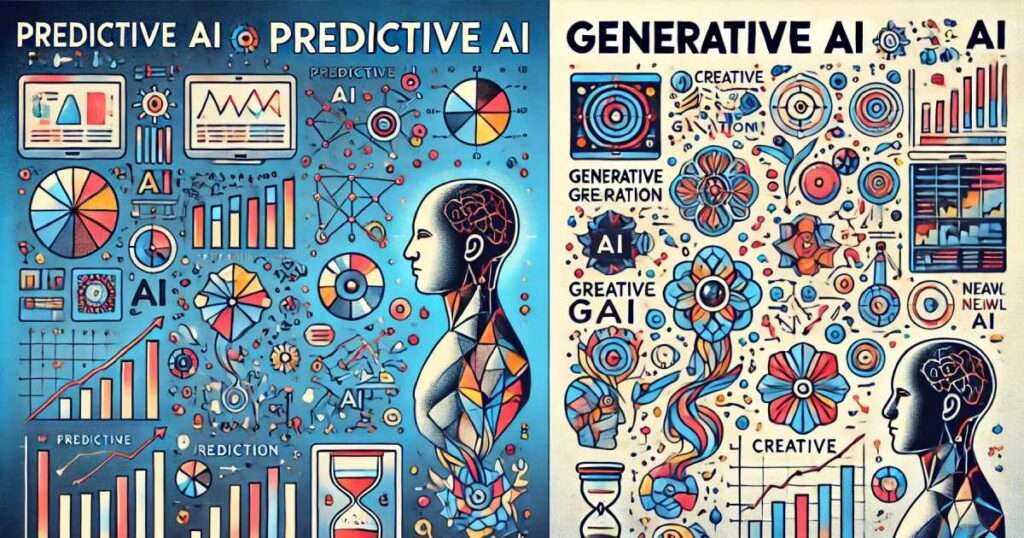
Advantages and Limitations of Predictive AI
- Advantages of Predictive AI: Predictive AI utilizes complex algorithms and machine learning to analyze historical data, offering invaluable insights across industries by accurately forecasting future outcomes, trends, and events. This enables organizations to make data-driven decisions, optimize strategies, and uncover patterns that traditional methods cannot, resulting in reduced risks, increased efficiency, and new growth opportunities.
- Limitations and Challenges of Predictive AI: The accuracy of predictive AI relies heavily on the quality, availability, and completeness of historical data. Limited or biased data can lead to inaccurate predictions, while models may struggle to anticipate unexpected disruptions. Additionally, ensuring transparency and comprehensibility in AI-driven predictions is critical, requiring continuous monitoring and refinement to maintain effectiveness as conditions evolve.
Advantages and Limitations of Generative AI
- Advantages of Generative AI: Generative AI revolutionizes content creation across design, art, and entertainment by automating workflows and boosting team efficiency. It enables faster, cost-effective production of high-quality, original content, pushing the boundaries of creativity and sparking conversations about job security within the creative industries.
- Limitations and Challenges of Generative AI: Despite its productivity benefits, generative AI presents challenges such as resource-intensive training, ethical concerns, and the complexity of collaboration. Leaders must balance creativity and control while addressing issues like bias, diversity, and the potential for workplace disputes over creative expectations and competition.
Aspect | Generative AI | Predictive AI |
Advantages | Revolutionizes content creation, automates workflows, enhances creativity, supports various industries (e.g., gaming, healthcare). | Offers accurate predictions, supports informed decision-making, optimizes business processes, enhances customer experiences. |
Limitations | Resource-intensive, ethical concerns, potential bias, lack of transparency in decision-making, risk of AI “hallucinations.” | Depends on the quality of historical data, can struggle with unexpected disruptions, requires continuous monitoring for accuracy. |
Applications | Content generation, creative industries, healthcare, gaming, marketing, and advertising. | Finance, marketing, e-commerce, supply chain management, manufacturing. |
Examples of Predictive AI
Forecasting Stock Market Trends
Predictive AI is an ideal fit for the finance industry. It analyzes historical market data, trends, and indicators, and then applies sophisticated machine-learning algorithms to past stock market data. With precision and accuracy, predictive AI models can forecast future stock prices and emerging market trends. Relying on these insights, investors and financial experts can make impressively astoundingly smart decisions on complex, high-stakes activities as when to buy, sell, or hold stocks.
Predicting Customer Behavior
When it comes to marketing, predictive AI is critical to evaluating customer data to predict their future decisions and behaviors. The AI can effortlessly inspect the complexities of past customer interactions like purchase history and browsing patterns. Armed with this wisdom, companies using predictive AI can foresee customer decisions, preferences, and interests or trends. The company can now personalize and customize its marketing strategies, tailor product offerings to customer preferences, and line up its strategies with anticipated customer preferences to raise revenue, value, and competitive strength.
Personalized Recommendations in E-commerce
Predictive AI perfects the user experience in e-commerce platforms by communicating accurate and personalized suggestions to users based on their browsing and purchase behavior. The company can make prophecies based on the customer’s experiences with products, and gain knowledge of their opinions as expressed to similar users. Predictive AI models can then align offerings, pricing, and other areas of interest to the specific user with amazing accuracy and precision. They can delight customers with innovative products at reduced prices, for example making them irresistible and raising revenue. They can upsell and cross-sell offerings successfully at the exact right time for the customer and boost sales effortlessly.
Predictive AI Applications and Use Cases
Finance and Banking
While generative AI in banking is widely used, predictive AI has increased its power and importance in the finance and banking arenas, leveraging historical data and complex, relevant algorithms to forecast market trends, stock prices, and investment opportunities. It supports risk assessment by closely examining transaction patterns and credit histories, improving the effectiveness of fraud detection and proactive prevention. Predictive AI models help speed the often frustrating and complex processes for loan approval and portfolio management. Now both businesses and clients can feel greater confidence in decision-making and in conducting accurate and efficient financial decision-making.
Retail and E-commerce
Predictive AI has boosted the capabilities needed for personalized customer experiences in retail and e-commerce. By taking a close and accurate look at past purchasing decisions and browsing behavior patterns, predictive AI can proactively identify customer preferences and create personalized, attractive product recommendations.
Predictive AI has made possible leaps forward in inventory management by predicting demand trends, avoiding inefficiencies like stockouts, and improving overall supply chain activities. These solutions yield customer satisfaction, drive sales, and streamline retail logistics to an amazing degree, creating an environment of greater productivity while enhancing revenue.
Manufacturing and Supply Chain Management
Predictive AI is responsible for history-making progress in manufacturing and supply chain management. Enterprises can now quickly and precisely optimize production schedules, resource allocation, and efficiency of logistics. Predictive AI preempts equipment maintenance needs, communicating them in advance, obviating downtime, and spurring operational efficiency to new levels.
With its ability to speed analysis of real-time data from sensors and monitors, predictive AI helps address equipment failures proactively, before they can cause damage. This approach minimizes disruptions, boosts productivity, and keeps maintenance costs under control. Manufacturing quickly recognized the value of predictive AI and continues to incorporate it into the manufacturing and supply chain arenas.
Generative AI Applications
Healthcare
We needn’t look far to find high-hope and high-value healthcare applications for generative AI. Impressive leaps in sophisticated medical imaging; drug research and progress in treating stubborn diseases; breakthroughs in genetic insight and exciting personalized treatment plans are emerging swiftly. Physicians and researchers now have AI resources for creating realistic medical images, diagnosing conditions accurately and swiftly, and innovating improved surgical planning and techniques.
Generative AI models are supporting new drug design by simulating molecular structures and interactions speeding headway. This has to potential to benefit countless victims of global diseases that have afflicted the human race for thousands of years. AI is also helping researchers improve their detection of emergent diseases and learn from the recent Covid-19 pandemic. The potential of GenAI to synthesize patient data allows for tailoring strategies for treating cancers and other conditions based on individual health profiles.
Gaming and Entertainment
The limitless creativity that GenAI can produce is revolutionizing the gaming and entertainment industries. Generative AI brings into being astounding immersive experiences, lifelike environments, characters, and animations. The innovations in gameplay and visual effects are helping the industry grow into fresh markets and acquire millions of new players.
Content creation too is a rich field for GenAI to produce scripts, dialogue, and narratives, adding fascinating depth to video games and movies. Additionally, chatbots powered by generative AI yield dynamic new interactions among players and game characters, improving the interactive experience and attracting player engagement and delight.
Marketing and Advertising
The power of generative AI is revolutionizing marketing and advertising strategies by developing exciting, convincing content, visuals, and designs. The technology quickly and automatically comes up with compelling ideas for smart ad copy, persuasive product descriptions, and convincing social media posts that attract and engage target audiences.
Progress in graphic design continues to amaze the industry with unique logos, exceptionally original visuals, and branding elements that work to attract new audiences and build current customer loyalty. The ability to personalize content at scale also charms and appeals to customers and aligns with various consumer tastes and affinities, spurring revenue and more productive marketing campaigns.
Implications and Ethical Considerations
1- Potential Impact on Jobs and Employment
Both generative and predictive AI have massive potential to influence the landscape of work and jobs. While predictive AI’s ability to automate routine and menial tasks has prompted concerns and disputes about job displacement, generative AI takes a different tack transforming creative occupations by automating content creation. Both of these AI technologies have caused anxiety in the workforce which enterprises must address. As generative and predictive AI grows more sophisticated, companies need to be aware of their workforce needs and the feelings of their employees. Such tactics as training for upskilling, and creating new roles that embrace AI capabilities of AI can help employees find their ground as the workplace changes around them and take advantage of new career opportunities.
2- Data Privacy and Security Concerns
Data privacy is one of the most controversial and critical aspects of both generative and predictive AI. Predictive AI uses volumes of historical data, which causes altercations about data privacy, confidentiality, and security breaches. Generative AI also requires access to data to create content. Human writers and artists are naturally concerned about the integrity of their original creative output. There are also risks of sensitive or personal information being exposed and publicly used without following proper legal processes and regulatory rules which are becoming more complex. Implementing robust measures to protect data, enforcing regulatory compliance, and ensuring transparent data practices are vital to preclude concerns and evade extended costly legal procedures.
3- Bias and Fairness Issues
The challenge of bias invading AI is nothing new. However, the swift emergence of generative AI has accelerated and intensified concerns at a rate greater than anticipated. Now, rather than anxiety over “a bit of bias” appearing here and there, enterprise leaders are tasked to prevent bias from assaulting their corporate cultures and taking over.
Such phenomena as “woke” political bias, gender-based, or race-based bias are sneaking into an output like ChatGPT, and critics have picked up on the risks.
Leaders must ensure that generative AI does not create toxic belief systems or habitual communication and language in the organization. Corporate and human values need to be encouraged, instilled, and overseen, and the issue must be brought out in the open, with users on alert for signs of bias and the subtle manifestations of it that can hurt people.
Bias in AI algorithms is a substantial ethical concern in both generative and predictive AI. Predictive AI’s reliance on historical data may preserve and maintain biases from the past, leading to unfair evaluations or hiring and promotional decisions.
Training generative AI on biased or prejudicial data content can become a real problem with consequences for customers, employees, and the bottom line when exposed to the public. Avoiding bias requires a commitment to diversity and fairness in training data, along with constant monitoring, and cautiously overseen transparency in model development to protect fairness and equity in AI applications.
Evaluation of Future Prospects
The future of generative AI and predictive AI holds immense potential within the landscape of AI trends. Generative AI’s creative capabilities can and will transform content creation and artistic expression, taking the organization into new terrain. Predictive AI’s ability to forecast trends could support businesses in making informed decisions raising revenue and operational efficiency to unprecedented levels. As these technologies advance, addressing ethical challenges openly and transparently while committing to responsible and honest uses will play a critical role in shaping AI’s future and trajectory.
Organizations, and indeed all users of AI, should be aware of the “Sorcerer’s Apprentice” phenomenon and shoulder responsibility for the consequences that these powerful technologies can bring with them that affect the entire human race.
Conclusion
In this exploration of generative AI and predictive AI, we’ve delved into their definitions, workings, applications, advantages, limitations, and future implications. Generative AI is focused on creating new content from images to text to music, while predictive AI turns to leveraging historical data to forecast future trends.
Both have almost incalculably diverse applications across industries, from healthcare to marketing, entertainment, manufacturing, and countless others. However, the success and ubiquity of these types of AI raise complex concerns, including bias, privacy, job displacement, accuracy, and security. The responsibilities that come with the opportunities are critical, and leaders must immerse themselves in the challenges of AI when they commit to implementing it.