What is AI Governance?
AI Governance refers to the core principles or framework on which AI systems and tools are built to make them ethical, transparent, and unbiased. With the onset of so many AI systems hitting the market designed to enhance efficiency and decision-making, responsible AI governance aims to establish a framework that ensures the ethical development and deployment of AI technologies, significantly increasing the importance of robust AI governance.
The Australian government incorporated artificial intelligence to calculate welfare payments, leading to incorrect debt notices sent to thousands of citizens. This further led to emotional and financial distress. As a result of this disaster, the Australian government received widespread backlash and lawsuits. The government ended up paying a settlement fine of over $1.2 billion. Such instances highlight the need for a safety net that AI governance provides, eliminating risk for organizations trying to integrate or even develop new AI systems.
Artificial intelligence (AI) governance is crucial in ensuring AI technologies’ responsible and ethical development. Humans design AI systems and are vulnerable to human biases and errors. The AI governance policies and practices ensure unbiased decision-making through AI solutions. Only through AI governance of the systems can processes and algorithms be monitored, evaluated, and updated continuously to prevent flawed decision-making.
AI Governance is not one of those compliance duties that are implemented once and then forgotten about. As AI models and machine learning systems evolve with new data, the AI governance framework is going to become table stakes when incorporating new AI solutions.
AI solutions are always going to be a two-edged sword with not just working in the capacity of an operator, helping you automate your daily tasks, but also capable of giving you skewed data or suggestions, which can have negative repercussions. Thus, AI governance becomes that overwatch that keeps everything under control and safe for end users.
AI Governance Frameworks and Standards
As organizations increasingly adopt AI technologies, the importance of robust governance frameworks cannot be overstated. Effective AI governance ensures that development and deployment processes align with ethical standards and regulatory requirements, fostering trust in AI and ensuring accountability.
Establishing an AI Governance Committee, a dedicated group comprised of representatives from various departments, can oversee AI governance and ensure alignment with the organization’s values and regulatory standards.
International frameworks and industry best practices provide essential guidelines for organizations to navigate this complex landscape. For instance, the OECD AI Principles emphasize human-centered values, fairness, transparency, robustness, and accountability, serving as a foundational reference for many national and organizational AI strategies.
Similarly, the UNESCO Recommendation on the Ethics of AI sets a global standard for ethical AI practices, ensuring that systems respect human rights and environmental sustainability. By adhering to these frameworks, organizations can create tailored governance strategies that not only comply with regulations but also promote Responsible AI practices that resonate with stakeholders. Building upon these established foundations, enterprises must then translate these broad principles into concrete actions.
AI Governance Strategies for AI Models
To truly harness the benefits of AI while mitigating potential risks, enterprises need tailored governance strategies that avoid legal risks and go beyond simply acknowledging international standards. These strategies set ethical boundaries and must directly align AI initiatives with business objectives, ethical expectations, and evolving regulatory requirements. A holistic strategy should encompass several key elements:
- Policy Creation: Develop clear, well-documented principles and guidelines addressing ethical AI use and legal compliance for every phase of AI system management, including development, testing, deployment, and monitoring.
- Oversight Structures: Establish specific roles and committees to oversee AI-related activities throughout the organization, ensuring accountability and alignment with defined business use cases.
- Risk Management: Implement protocols for continuous monitoring and mitigation of risks such as data privacy issues, biases in AI models, and security vulnerabilities, leveraging frameworks like TRAPS (Trusted, Responsible, Auditable, Private, Secure) to ensure agentic security and ethical development.
- Ethical Decision-Making Frameworks: Adopt internal policies or recognized external standards to support clear and accountable ethical practices throughout the AI lifecycle.
- AI TRiSM Implementation: Implement AI Trust, Risk, and Security Management (AI TRiSM) to proactively manage risks associated with AI solutions, continuously monitor model performance, and ensure robust data security measures.
- Generative AI Governance: Address the unique challenges posed by Generative AI—such as AI Hallucination and the need for domain-specific LLMs or even small language models—by implementing specific safeguards and monitoring mechanisms, ensuring trust is maintained while leveraging the technology’s power.
By proactively implementing these strategies, enterprises can build a foundation for trustworthy and responsible AI, ensuring that their AI initiatives drive business value, uphold ethical standards, and comply with evolving regulatory landscapes.
Key Components of AI Governance
Effective AI governance involves several key components that work together to ensure the responsible development and deployment of AI systems. Responsible AI is also crucial in ensuring the ethical deployment of AI systems and mitigating risks associated with their use. These components include:
- Accountability and Responsibility: Accountability in AI governance is another important pillar that ensures the responsible use of AI technologies. As these technologies become more integrated into decision-making processes, determining who is responsible for any harm done becomes more complex. In 2018, one of the autonomous vehicles operated by Uber was met with an accident, killing a pedestrian in Tempe, Arizona. This raised serious concerns about the artificial intelligence system’s accountability, specifically in this case, regarding who should be held responsible for the loss of human life.To build robust accountability structures, companies can take several steps:
- Establish Clear Chains of Responsibility: Define unambiguous roles and responsibilities for AI decisions.
- Implement Shared Accountability Models: Spread responsibility across multiple stakeholders, including developers and business leaders.
- Develop Dedicated AI Oversight Teams: Form specialized teams to monitor and document AI-related decision-making.
- Ethics and Fairness: Developing and implementing ethical guidelines and principles is essential to ensure that AI systems are fair, unbiased, and respectful of human rights and dignity. This helps prevent the reinforcement of societal inequalities and promotes equitable outcomes.
- Transparency and Explainability: Transparency and explainability are two of the key pillars of AI Governance. When companies can’t really explain the underlying working of the algorithms or decision-making, it can lead to distrust among users and operations can be impacted too. One of the healthcare institutions implemented a new AI technology that would determine which patients get prioritized over others. However when the questions arose about the underlying logic used to prioritize, the company couldn’t come up with a justified answer. This led to patients losing trust in the organization and regulatory scrutiny by the authorities.
- Risk Management: Identifying, assessing, and mitigating risks associated with AI system development and deployment is a critical aspect of AI governance. This includes addressing risks related to data quality, security, and bias to ensure the reliability and safety of AI systems.
- Global AI Governance: Establishing global standards and frameworks for AI governance is necessary to ensure consistency and coordination across borders and industries. This helps create a unified approach to AI governance, promoting ethical and responsible AI development on a global scale.
- Data Privacy and Security: Every AI technology in the market heavily relies on user data sets, which it eventually leverages to improve. It becomes the sole factor behind the success or failure of an AI model. Thus, protecting data is an important pillar of AI governance. One of the best examples of this is the 2016 Facebook Cambridge Analytica scandal, where the personal data of millions of users was accessed without their consent. This led to the creation of psychological profiles for political targeting during the US presidential elections of 2016. This massive data breach led to the shutting down of Cambridge Analytica.
- Bias & Fairness: Creating an AI system that is unbiased and fair becomes extremely difficult when the system inadvertently reinforces societal inequalities that already exist. In 2018, Amazon came up with an AI recruitment tool that automated the process of finding and narrowing down candidates’ profiles in accordance with the job profile. As it turns out, the system was biased towards male candidates. The reason behind this was that the algorithm was trained on resumes submitted over a span of the last 10 years, predominantly from male candidates, which reflects the imbalance in the tech industry.
- Compliance: Compliance is another big challenge to sustain AI governance since it is continuously evolving and any non-compliance on the organization’s part comes with heavy penalties and scrutiny.
GDPR compliance came into effect in May 2018, which stated that any organization that processes the personal data of EU citizens, regardless of where the organization is located, has to comply. This regulation has profound implications for organizations that rely on personal data for training and decision-making purposes.
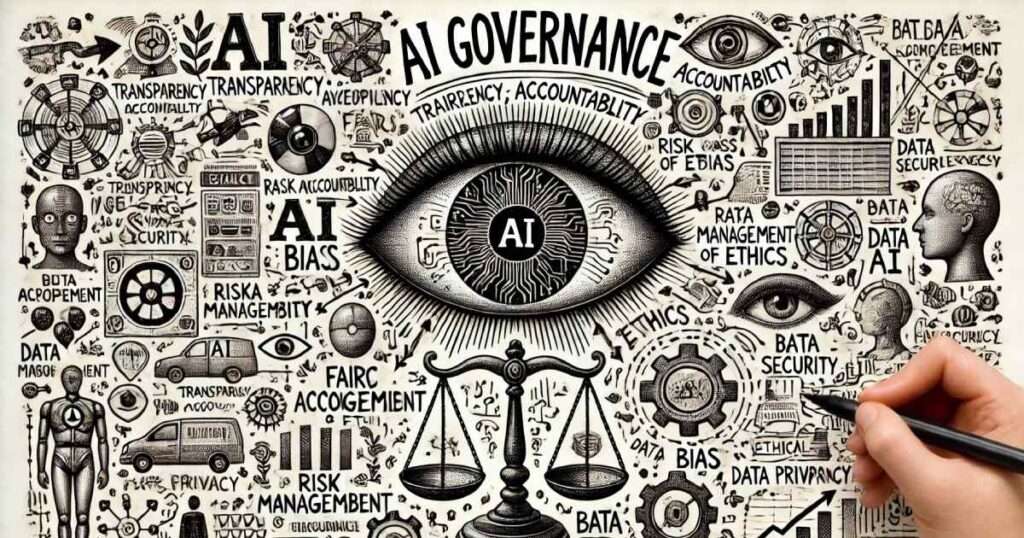
3 Key Challenges of AI Governance
Dynamic Regulatory Landscape
The regulatory landscape of AI is constantly changing and evolving. Each country is creating its own unique policies and regulations to navigate this upcoming new technology. Being globally compliant with the regulations is complex and also being able to anticipate future requirements further adds to the complexity.
Implementing effective governance practices is crucial to address these challenges, ensuring that organizations allocate adequate resources, define roles, and develop comprehensive frameworks that address compliance, ethical considerations, and transparency.
Fast-Paced Evolution of AI Technologies
The new technologies, like Gen AI and foundation models, are emerging at a fast pace. These technologies create complex and unpredictable outputs, making incorporating transparency and unbiased decision-making difficult. When companies cannot keep up with the new trends, they miss opportunities and are exposed to the risk of deploying unregulated AI solutions.
Lack of Understanding and Expertise
The need for a subject matter expert is of grave importance when it comes to building a framework for AI governance. Overcoming this challenge requires investing in education and training to develop the necessary skills and knowledge.
Metrics and KPIs for Measuring AI Governance Effectiveness
To ensure that AI governance strategies are effective, enterprises must establish clear metrics and Key Performance Indicators (KPIs) to measure their impact. Here are some key metrics and KPIs to consider:
- Compliance Rate: Track the percentage of AI systems that comply with established policies and regulatory standards.
- Risk Mitigation Success: Measure the number of identified risks that are successfully mitigated through governance processes.
- AI Model Performance: Monitor the accuracy and reliability of AI models, ensuring they meet predefined standards.
- Transparency and Explainability: Evaluate the extent to which AI decisions are transparent and explainable to stakeholders.
- Audit and Compliance Costs: Track the financial impact of maintaining compliance and conducting audits to ensure cost-effectiveness.
- Innovation and Efficiency Gains: Measure the improvements in business processes and innovation driven by AI governance strategies.
By regularly assessing these metrics, organizations can refine their AI governance frameworks to better align with business objectives and ethical standards, ultimately enhancing the overall effectiveness of their AI initiatives.
AI Risk Management and Compliance
Artificial intelligence offers immense potential, but without proper risk management and compliance, organizations are essentially walking a tightrope without a safety net. Failing to address AI risks can lead to significant financial losses, reputational damage, and legal repercussions.
What happens when AI governance goes wrong? Here are a few cautionary tales:
- Zillow’s Algorithmic Home-Buying Disaster: Zillow Offers, the company’s iBuying program, used algorithms to estimate and forecast real estate prices with the intent to buy and flip properties for a profit. However, their models underestimated market growth, leading to significant losses. Zillow ultimately had to wind down its Zillow Offers operations, write down millions, and cut 25% of its workforce15.
- Tay, Microsoft’s Chatbot Disaster: Microsoft’s AI chatbot Tay was shut down after it began posting offensive and racist tweets. The incident underscored the importance of careful monitoring and ethical guidelines for AI systems.
- IBM Watson for Healthcare: IBM Watson was touted as the driver for a revolution in medicine. The business unit was recently sold off for parts for $1B, with a loss of at least $5B just in acquisitions.
To avoid similar pitfalls, organizations must prioritize AI risk management and compliance. This includes implementing robust data governance frameworks, conducting regular AI model validation and monitoring, and adhering to relevant regulations like GDPR. By taking these steps, organizations can harness the power of AI responsibly and avoid costly mistakes.
Implementing AI Governance in an AI System
Implementing effective AI governance requires a multi-faceted approach that integrates ethical considerations, compliance measures, and continuous monitoring. A crucial element is establishing AI ethics boards and empowering compliance teams to systematically identify and mitigate ethical risks. These bodies should have the authority to review AI proposals, recommend changes, and ensure alignment with ethical standards.
Best practices for responsible AI deployment include embedding responsible AI principles into the organizational structure and fostering a culture of Responsible AI awareness through continuous training. Collaboration between departments and defined roles are vital for AI governance. Organizations should also make AI systems explainable by ensuring transparency in every phase of development. Here’s how companies are effectively implementing AI governance:
- Vodafone Idea: Enhancing AI Governance through Data Governance – Initially, Vodafone Idea struggled with disparate data sources affecting AI model accuracy. They consolidated data into a single lake, improving data quality and AI performance. This transformation enabled better scalability and collaboration, enhancing decision-making and customer experience.
- IndianOil: Driving Digital Transformation and AI Governance – Before, IndianOil lacked structured AI governance, limiting AI leverage. They created a Strategic Information Systems group to drive digital transformation and implement AI governance. This led to improved oversight, ensuring AI projects aligned with corporate goals and ethical standards and enhancing operational efficiency.
- BMW: Establishing Responsible AI Practices – Prior to “Project AI” competence centers, BMW faced challenges in managing AI data responsibly. These centers improved data management and ensured responsible AI practices. This transformation facilitated better collaboration and innovation while maintaining high ethical standards.
Continuous monitoring and adaptive governance are essential for maintaining AI system reliability and effectiveness. Real-time data analysis and feedback loops enable organizations to track AI performance and identify issues like data drift. AI TRiSM should be implemented to manage risks associated with AI solutions, monitor model performance, and ensure data security. Adaptive governance involves regular audits and assessments to evaluate performance and adjust policies as needed.
Future of AI Governance and Emerging Trends
The rapid evolution of generative AI and automation is reshaping industries and societies alike, demanding a new era of AI governance. As AI systems become increasingly sophisticated and integrated into critical infrastructure, the need for clear ethical guidelines and regulatory frameworks becomes paramount.
We stand at a pivotal moment where proactive measures are essential to ensure that AI benefits humanity while mitigating potential risks. This requires a collaborative effort involving governments, industry leaders, and experts to establish standards and best practices that foster trust and transparency in AI technologies.
The emergence of Artificial General Intelligence (AGI) and edge AI introduces new complexities to the AI governance landscape. AGI, with its potential to perform any intellectual task that a human being can, raises profound ethical and safety questions that must be addressed through careful planning and oversight.
Edge AI, which brings AI processing closer to the data source, presents unique challenges related to data privacy and security. Adaptive governance models are needed to address these emerging technologies, ensuring that they are developed and deployed responsibly and in alignment with human values.
Global AI governance requires international cooperation and a shared commitment to ethical principles. Policy recommendations include establishing international standards for AI development and deployment, promoting transparency in algorithms, and ensuring accountability for AI-driven decisions. Collaboration among nations is crucial to harmonize regulations and prevent a fragmented approach that could hinder innovation or create loopholes for unethical practices. By prioritizing these elements, we can collectively shape a future where AI is a force for good, benefiting all of humanity while safeguarding against potential harm.